Virtual screening of compounds derived from Garcinia pedunculata as an inhibitor of gamma hemolysin component A of Staphylococcus aureus
Abstract
With the emergence of multi-drug resistant pathogens at alarming frequency, there has been an increase interest in the development of novel drugs from natural resources. The use of higher plants and preparations made from them to treat infections is a longstanding practice in a large part of the population, especially in the developing countries, where there is dependence on traditional medicine for a variety of ailments. The virtual screening method was used in this study to analyze the docking and inhibitory activities of some natural bioactive compounds present within Garcinia pedunculata against hemolysin toxin of Staphylococcus aureus, gamma-hemolysin component A hlgA. The study resulted in identifying compounds 1,3,6,7-tetrahydroxyxanthone and garcinone D with high binding affinity towards the target protein revealing them as potent inhibitors that could be further used to create new drug source in the treatment of staphyloccocal infections.
Introduction
Natural products have historically and continually been investigated for promising new leads in pharmaceutical development. They have been looked upon as a potential source to avail alternate drug source due to rise in multiple drug resistance to antibiotics in human in recent years (Marvin et al., 1975; Church et al., 2006). Screening of the various bioactive compounds in a particular plant through computer based approaches has diminished the time-limit utilized during preclinical studies. The use of computational techniques as virtual screening in drug discovery and development process along with chemical and biological analysis has improved the effectiveness and efficiency of the process. Langer and Wolber (2004) had described virtual screening as the computational approach of filtering of large databases or libraries of candidate compounds based on physicochemical, pharmaceutical, ADMET/PK (pharmacokinetic) properties that permit the selection of series of compounds to be tested for biological activity. In another words, in silico modeling is used to significantly minimize time and resource requirements of chemical synthesis and biological testing.
Staphylococcus aureus is a common host in human flora that asymptomatically colonizes one in three healthy individuals and is the second cause of hospital and community-acquired infections worldwide due to increased drug resistance and as a result morbidity (David and Daum, 2010; DeLeo et al., 2010; David et al., 2011). The Gamma-toxins of S. aureus has been proposed to play a role in the pathogenesis of toxic shock syndrome (TSS) together with toxic shock syndrome toxin 1 (TSST-1), since this hemolysin is very frequently found in TSS isolates (Clyne et al., 1988). S. aureus gamma-hemolysins (HlgA, HlgB and HlgC) and Panton-Valentine leucocidins (LukS-PV and LukF-PV) are bi-component toxins forming a protein family. Toxins HlgA + HlgB and HlgC + HlgB induce permeabilization of model membranes by forming pores via oligomerisation causing subsequent hemolysis (Ferraras, 1998). Among them, HlgA and HlgC, two of the three related proteins expressed from the staphylococcal γ-hemolysin locus are the ones to bind first to cell membranes and then subsequently act on the lipidic vesicles, erythrocytes and polymorpho-nuclear cells (Meunier et al., 1997). HlgA (class S) associates with HlgB (class F) thus forming an AB toxin in strains producing both gamma-hemolysins and leukocidins. HlgA and LukF-PV can also form a complex. Thus it is assumed that selective inhibition of any one of the related protein will prevent the formation of the required toxic complexes for hemolysis.
This study was performed to analyze the inhibitory effects of certain naturally occurring compounds of Garcinia pedunculata ex Bach-Ham on gamma-hemolysin component A hlgA of S. aereus, their interaction details and effectiveness in inhibiting the target protein. In this study 3D structure of hlgA was used as receptor in-silico inhibition analysis against a created ligand dataset of G. pedunculata compounds. Each docking experiment was analyzed carefully for finding correct orientations of ligands with receptor binding site.
Materials and Methods
Target identification
The three dimensional structure of gamma-hemolysin component A hlgA of S. aereus subsp. aureus MW2 was obtained from RCSB Protein DataBank (http:www.pdb.org). Its PDB id is 2QK7 (Robin et al., 2008), chain A, with Uniprot accession number as P0A074 (HLGA_STAAU). It is a 309 bp sequence with a molecular mass of 34,956 dalton. Its resolution is 2.40 A° produced through X-ray diffraction technique. The protein sequence is given below in FASTA format:
>sp|P0A074|HLGA_STAAU Gamma-hemolysin component A OS = S. aureus GN=hlgA PE=1 SV=1
MIKNKILTATLAVGLIAPLANPFIEISKAENKIEDIGQGAEIIKRTQDITSKRLAITQNIQFDFVKDKKYNKDAL
VVKMQGFISSRTTYSDLKKYPYIKRMIWPFQYNISLKTKDSNVDLINYLPKNKIDSADVSQKLGYNIGGNFQ SAPSIGGSGSFNYSKTISYNQKNYVTEVESQNSKGVKWGVKANSFVTPNGQVSAYDQYLFAQDPTGPAARD
YFVPDNQLPPLIQSGFNPSFITTLSHERGKGDKSEFEITYGRNMDATYAYVTRHRLAVDRKHDAFKNRNVTVK
YEVNWKTHEVKIKSITPK
Detection of cavities and binding sites
All the cavities in the target structures were detected using Q-site finder (Laurie and Jackson, 2005) and cavities detection option of Molegro docker within both the chains.
Preparation of the ligand dataset
The fruit of G. pedunculata is considered to have high medicinal properties. As such the compounds identified within the fruits of G. pedunculata were collected from various literatures (Sahu et al., 1989) to create the ligand dataset for the current study. The various biochemical properties were analyzed in Chemspider (www.chemspider. com) and PUBCHEM (http:pubchem.ncbi.nlm.nih. gov/). The 2D structures obtained were redrawn using MDL-ISIS Draw (www.mdli.com) and Chemsketch (http:www.acdlabs.com) and converted to 3D using Chem3Draw ultra and saved in .mol2 file. Energy minimization and geometrical optimization were done using MM2 (Merck Molecular mechanics) (Lii et al., 2009) calculation of Chemoffice while the lead optimization of the selected compounds, the druglikeness score and bioactivity of the lead molecules was done through in silico Lipinski filter (Lipinski et al., 2001) with the help of software Molinspiration drug-likeness score online (www.molinspiration.com) and the results were compared with standard drug ciprofloxacin.
Molecular docking studies
Docking and inhibition analysis of a protein-ligand complex was done by measuring the total electrostatic potential spent during protein-ligand interactions through Molegro Virtual Docker (MVD). It was used to dock compounds to generate an ensemble of docked conformations and each scoring function was applied to generate classes based on the obtained dock scores followed by ranking the best conformations. The grid resolution was set at 0.30 Angstrom for grid generation, RMSD Threshold at 1.0 return of multiple poses for each run at 10 and a 15 Angstrom radius from the template as the binding site. A population size of 50, maximum iteration of 2000, scaling factor of 0.50, cross-over rate of 0.90 and a variation based termination scheme for parameter settings were used as the docking parameters for obtaining the final docked structures. All the water molecules and co-crystallized ligand were removed from the target protein prior to docking. All chains and all detected cavities within the chains were taken for docking analyses. Polar hydrogens were added to the receptor, Kollaman charges were assigned and solvation parameters were added and for the inhibitors, charges of the Gasteiger type were assigned. The best ligand-receptor structure from the docked structures was chosen based on lowest energy and minimal solvent accessibility of the ligand.
Results
A total of 10 cavities were detected in the target protein 2QK7.pdb, Chain A. The secondary structure rendered receptor, 1,3,5,7-tetrahydroxyxanthone and garcinol D was seen to be the best inhibitors of the target protein post docking and inhibition process. Their inhibitory activity was found to be better than the control ligand ciprofloxacin in terms of H-bond interaction, Moldock and Rerank score as seen in Molegro Docker Platform. Among the multiple binding sites, the best cavity and ligand-receptor affinities (scoring functions) was based on the least but the best rerank score and the highest MolDock score The details of the bioactive compounds considered for the docking execution in this study is given in Table II while the ligands with the best scores are given in Table III.
Table I: Details of two active site cavities with target receptor protein used by the ligands for docking
Cavity | Volume (A°) | Binding site coordinates | Amino acid residues |
---|---|---|---|
2 5 |
161 111 |
Min: -7,-34,3; Max:8,-20,18 Min: -16,-63,20; Max: -3,-49,33 |
635 CG Leu 95-830 CD Pro121 680CA Asp101-1131N-Phe163 |
Docking 1,3,6,7-tetrahydroxyxanthone into 2QK7.A
In UCSF Chimera (Pettersen et al., 2004) is given in Figure 1. Out of these, docking was seen to take place only within two active sites, cavity 2 and 5 the details of which is given in Table I.
Figure 1: 3D-structure of 2QK7.A.pdb of gamma-hemolysin component A of Staphylococcus aureus subsp. aureus MW2 rendered in UCSFChimera software
Based on the binding compatibility (kcal/mol) with the receptor, 1,3,5,7-tetrahydroxyxanthone and garcinol D was seen to be the best inhibitors of the target protein post docking and inhibition process. Their inhibitory activity was found to be better than the control ligand ciprofloxacin in terms of H-bond interaction, Moldock and Rerank score as seen in Molegro Docker Platform. Among the multiple binding sites, the best cavity and ligand-receptor affinities (scoring functions) was based on the least but the best rerank score and the highest MolDock score The details of the bioactive compounds considered for the docking execution in this study is given in Table II while the ligands with the best scores are given in Table III.
Table II: Details of compounds from Garcinia pedunculata taken for docking and inhibition analysis along with ciprofloxacin
Name | IUPAC Name | Smiles Sting | Hydrogen acceptor count | Hydrogen donor count | Rule of 5 violations |
---|---|---|---|---|---|
Ciprofloxacin (C17H18FN3O3) |
1-cyclopropyl-6-fluoro-4-oxo-7-(piperazin-1-yl)-1,4-dihydro-quinoline-3-carboxylic acid | OC(=O)C1=CN(C2CC2)C2=CC(N3CCNCC3)=C(F)C=C2C1=O | 6 | 2 | 0 |
Garcinol | (1S,3S,4aR,6S,7R,8aR,9R,9aS,10aS)-dodeca hydro-1H-xanthene-1,3,6,7,9-pentol | OC1C3C(OC2C1C(O)CC(O)C2)CC(O)C(O)C3 | 6 | 5 | 0 |
1,3,6,7-tetrahydroxyxanthone (C13H8O6) |
1,3,6,7-Tetrahydroxy-9H-xanthen-9-one | C1=C(C=C2C(=C1O)C(=O)C3=CC(=C(C=C3O2)O)O)O | 6 | 4 | 0 |
Garcinone D (C24H28O7) |
1,3,6-Trihydroxy-8-(3-hydroxy-3-methylbutyl)-7-methoxy-2-(3-methyl-2-buten-1-yl)-9H-xanthen-9-one | O=C1C4C(OC3C1C(O)C2C(OCO)(C2)C(O)(C)C)C3)CCC(O)C4C\C=C(/C)C | 7 | 4 | 0 |
Garcinone C (C23H26O7) |
1,3,6,7-Tetrahydroxy-8-(3-hydro-xy-3-methyl butyl)-2-(3-methyl-2-buten-1-yl)-9H-xanthen-9-one | O=C1C3C(OC2CC(O)=C(C(O)C12)C\C=C(/C)C)C=C(O)C(OC)C3CCC(O)(C)C | 7 | 5 | 1 |
Camboginol (C38H50O6) |
(1S,3E,5R,7R)-3-[(3,4-Dihydroxy-phenyl)(hydroxy)methylene]-1-[(2S)-2-isopropenyl-5-methyl-4-hexen-1-yl]-6,6-dimethyl-5,7-bis(3-methyl-2-buten-1-yl)bicyclo[3.3.1]nonane-2,4,9-trione | O=C1[COO]2(C(=O)/C(C(=O)[CO]1(C[COOH](C\C=C(/C)C)C2(C)C)C[COOH](C(=C)\C)C\C=C(/C)C)=C(/O)C3CCC(O)C(O)C3)C\C=C(/C)C | 6 | 3 | 1 |
Table III: Energy score and H-bond interactions of the ligands with the target protein Chain A of 2QK7.pdb
Ligand | MolDock Score(Kcal/mol) | Rerank Score(Kcal/mol) | Hbond |
---|---|---|---|
2QK7 | 2QK7 | 2QK7 | 2QK7 |
Garcinol | -92.7767 | -84.0364 | -14.6613 |
1,3,6,7-tetrahydroxyxanthone | -86.7767 | -75.4433 | -10.7390 |
Garcinone D | -83.3073 | -78.7941 | -11.6320 |
Garcinone C | -105.8080 | -80.0659 | -8.0126 |
Camboginol | -108.3073 | -83.7695 | -8.8784 |
Ciprofloxacin | -106.7670 | -73.9882 | -1.9906 |
Docking 1,3,6,7-tetrahydroxyxanthone into 2QK7.A
Docking simulation of 1,3,6,7 tetrahydroxyxanthone into 2QK7 produced 5 clusters of conformers out of 10 docking runs. The binding energy of -86.8 Kcal/mol at 10th run had 6 hydrogen bond interactions at residues Lys97, Lys99, Gln107, and Ser119. The docking interactions between the ligand and the macromolecule are given in Table IV.
Docking garcinone D into 2QK7.A
Docking simulation of garcinone D into 2QK7 produced 3 clusters of conformers out of 10 docking runs in cavity 5. The binding energy of -83.3 Kcal/mol at 10th run had 6 hydrogen bond interaction at residues Asp101, Asn141, Tyr142, Ser162 and Gln169. The docking interactions between the ligand and the macromolecule are given in Table IV.
Table IV: H-bond interaction with the best ligands from G.pedunculata along with ciprofloxacin
Protein/Enzyme | Ligand/lead compounds | Amino acids | Distance (Angstrom) | Energy |
---|---|---|---|---|
2QK7 | CAVITY 2 | |||
1,3,6,7-tetrahydroxyxanthone | Lys 97 | 3.0 | -2.5 | |
Lys 99 | 2.4 | -1.2 | ||
Gln107 | 3.2 | -2.2 | ||
Gln107 | 2.9 | -2.5 | ||
Ser 119 | 3.5 | -0.7 | ||
Ser 119 | 3.0 | -2.5 | ||
CAVITY 5 | ||||
Garcinone D | Asp 101 | 2.4 | -2.5 | |
Asn 141 | 3.1 | -1.1 | ||
Tyr 142 | 3.1 | -2.5 | ||
Ser 162 | 3.0 | -0.5 | ||
Ser 162 | 2.5 | -2.5 | ||
Gln 169 | 3.1 | -1.6 | ||
Ciprofloxacin | Tyr 94 | 2.8 | -0.4 | |
Val 146 | 3.3 | 0.2 | ||
Val 146 | 3.6 | -0.3 | ||
Val 146 | 2.9 | -1.8 |
Docking ciprofloxacin into 2QK7.A
Docking simulation of ciprofloxacin into 2QK7 produced 5 clusters of conformers out of 10 docking runs in cavity 5. The binding energy of -106.8 Kcal/mol at 10th run had 4 hydrogen bond interaction at residues Tyr94 and Val 146. The docking interactions between the ligand and the macromolecule are given in Table IV.
Discussion
Docking and inhibition analysis exhibited that the complexes of protein-ligand interactions was in good agreement with experimental data, despite having different global conformations of the ligand. It was seen that more than one possible ligand conformation was stable within the spacious and symmetric active-site pores of the dockable targets. The final molecular simulations of the docked complexes provided molecular insight into the preferential binding of the inhibitors to the targets.
Staphylococcal gamma-hemolysins are bicomponent toxins forming a protein family with leucocidins and alpha-toxin (Ozawa et al., 1998). They are active as a combination of two similar proteins which form hetero-oligomers. Inhibition of one protein in the complex decreases the functionality of the whole complex system (Meunier et al., 1997). Two cavities were found to be responsible for ligand interaction within the protein. These sites could be the best possible binding sites to inhibit the gamma hemolysin component A.
The virtual screening method utilized in this study resulted in identifying compound 1,3,6,7-tetrahydroxyxanthone and garcinone D with high binding affinity towards gamma-hemolysin component A of S. aureus. The docked poses of these two compounds revealed more number of H-bond interactions than the registered drug ciprofloxacin. It was also found that the ligand molecules 1,3,6,7-tetrahydroxyxanthone and garcinone D satisfies the 'rule-of-5' and could be considered as potent inhibitors. This study reveals the importance of small molecules from various plant sources as docking agents. Further, work can be extended to study the receptor-ligand interactions experimentally and evaluation of their biological activity would help in specific isolation and effective treatment of diseases.
References
Church D, Elsayed S, Reid O, Winston B, Lindsay R. Burn Wound Infections. Clin Microbiol Rev. 2006; 19: 403–34.
Clyne M, De Azavedo J, Carlson E, Arbutnott J. Production of gamma-hemolysin and lack of production of alpha-hemolysin by Staphylococcus aureus strains associated with toxic shock syndrome. J Clin Microbiol. 1988; 26: 535–39.
David MZ, Boyle-Vavra S, Zychowski DL, Daum RS. Methicillin-susceptible Staphylococcus aureus as a predominantly healthcare-associated pathogen: A possible reversal of roles. PLoS ONE. 2011; 6: e18217.
David MZ, Daum RS. Community-associated methicillin-resistant Staphylococcus aureus: Epidemiology and clinical consequences of an emerging epidemic. Clin Microbiol Rev. 2010; 23: 616–87.
DeLeo FR, Otto M, Kreiswirth BN, Chambers HF. Community-associated meticillin-resistant Staphylococcus aureus. Lancet 2010; 375: 1557–68.
Ferreras M, Höper F, Dalla Serra M, Colin DA, Prévost G, Menestrina G. The interaction of Staphylococcus aureus bi-component gamma-hemolysins and leucocidins with cells and lipid membranes. Biochim Biophys Acta. 1998; 1414: 108-26.
Langer T, Wolber T. Virtual combinatorial chemistry and in silico screening: Efficient tools for lead structure discovery. Pure Appl Chem. 2004; 76: 991–96.
Laurie AT, Jackson RM. Q-SiteFinder: An energy-based method for the prediction of protein-ligand binding sites. Bioinformatics 2005; 21: 1908-16.
Lii JH, Gallion S, Bender C, Wikstrom H, Allinger NL, Flurchick KM, Teeter MM. Molecular mechanics (MM2) calculations on peptides and on the protein crambin using the Cyber 205. J Comp Chem. 1989; 10: 503-13.
Lipinski CA, Lombardo F, Dominy BW, Feeney PJ. Experimental and computational approaches to estimate solubility and permeability in drug discovery and development settings. Adv Drug Delivery Rev. 2001; 46: 3-26.
Marvin JA, Heck EL, Loebl EC, Curreri EL, Baxter CR. Usefulness of blood cultures in confirming septic complications in burn patients: Evaluation of a new culture method. J Trauma. 1975; 15: 657-62.
Meunier O, Ferreras M, Supersac G, Hoeper F, Baba-Moussa L, Monteil H, Colin DA, Menestrina G, Prévost G. A predicted beta-sheet from class S components of staphylococcal gamma-hemolysin is essential for the secondary interaction of the class F component. Biochim Biophys Acta. 1997; 12: 1326: 275-86.
Ozawa T, Kaneko J, Kamio Y. Essential binding of LukF of staphylococcal gamma-hemolysin followed by the binding of H gamma II for the hemolysis of human erythrocytes. Biosci Biotechnol Biochem. 1995; 59: 1181-83.
Pettersen EF, Goddard TD, Huang CC, Couch GS, Greenblatt DM, Meng EC, Ferrin TE. UCSF Chimera: A visualization system for exploratory research and analysis. J Comput Chem. 2004; 25: 1605-12.
Roblin P, Guillet V, Joubert O, Keller D, Erard M, Maveyraud L, Prevost G, Mourey L. A covalent S-F heterodimer of leucotoxin reveals molecular plasticity of beta-barrel pore-forming toxins. Proteins 2008; 71: 485-96.
Menestrina G, Dalla Serra M, Coraiola M, Viero G, Comai M, Potrich C, Ferreras M, Baba-Moussa L, Colin DA, Bhakdi S, Prévost G. Staphylococcus aureus bicomponent gamma-hemolysins, HlgA, HlgB, and HlgC can form mixed pores containing all components. J Chem Inf Model. 2005; 45: 1539-45.
Sahu A, Das B, Chatterjee A. Polyisoprenylated benzophenones from Garcinia pedunculata. Phytochemistry 1989; 28: 1233–35.
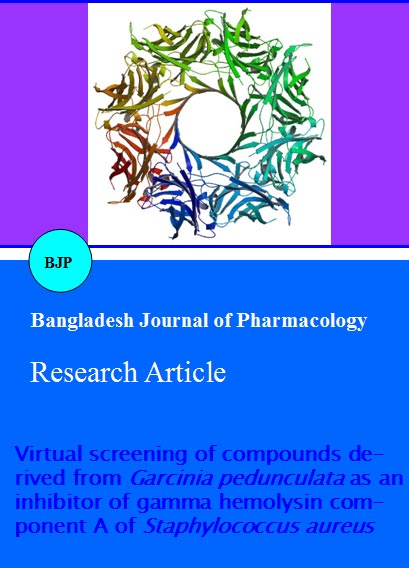