3D QSAR modeling of 4-nerolidylcatechol derivatives and virtual screening for identification of potent plasmodium inhibitor
Abstract
The present study was aim to develop a three dimensional quantitative structure-activity relationships (3D QSAR) model based on the structure of 4-nerolidylcatechol (IC50 = 0.67 µM), a novel plant derived Plasmodium inhibitor and its derivatives for identification of efficient antimalarial lead. A statistically validated Partial Least-Squares (PLS) based Molecular Field Analysis (MFA) model was built up using the training set of eight 4-nerolidylcatechol derivatives and their diverse conformers. A statistically reliable model with good predictive power (cross-validated correlation coefficient q2 = 0.769) was obtained. Hence, the generated model was used to screen a library of 30,000 compounds of chembridge database. Results of drug likeness prediction and ADMET study has suggested six compounds as potential antimalarial/plasmodial lead.
Introduction
Malaria is one of the deadly diseases causes due to the infection of Plasmodium spp and becomes a major public health problem of the world. Till date, malaria causing the death of 1-2 million people annually around the World and estimated reports of 300-500 million cases of Malaria (Bharati and Ganguly, 2013; Mohapatra et al., 1998; NVBDCP, 2010). Detection and report of drug resistance stains of malaria parasite has been treated as a major challenge to control malaria and leading to the continuous increases of malarial incidence (Crompton et al., 2014; Ghosh et al., 2000). Till date, not a single drug has been discovered for the complete eradication of all type Plasmodium spp. Therefore, development of new potential antimalarial candidate lead is a time demanding issue.
4-Nerolidylcatechol (4-NC, Figure 1) is a metabolite reported from Piper peltatum L. and Piper umbellatum L. (syn. Pothomorphe peltata (L.) Miq. and Pothomorphe umbellata (L.) Miq. The 4-nerolidylcatechol has been studied for strong antimalarial property including antioxidant and anti-inflammatory activities as per the data of previous studies. In a recent investigation, new derivatives of 4-NC were synthesized and experimentally validated for the inhibition of Plasmodium (Bagatela et al., 2013; Pinto et al., 2009; Rocha et al., 2011; Lima et al., 2012).
Figure 1: 2D structure of 4-nerolidylcatechol (IC50 = 0.67 µM)
Materials and Methods
Dataset preparation: A set of eight 4-nerolidylcatechol derivatives with their diverse experimentally known inhibitory activity (IC50) data was compiled from the literature. 4-nerolidylcatechol is a semi synthetic derivative of catechol known for its antimalarial activity. (Bagatela et al., 2013; Pinto et al., 2009; Rocha et al., 2011; Lima et al., 2012).
Compounds 2D structures were drawn using MarvinSketch v6.2 and Open Bable software was used for molecular file conversion purpose. Energy minimization for all the dataset compounds were performed using Discovery Studio v3.1 at CHARMM module (Arooj et al., 2011). Prediction of physiochemical properties of the dataset compounds such as hydrogen bond donor, hydrogen bond acceptor, Alogp, Molecular Weight (In Dalton), etc were also computed for drug likeness study (Gogoi et al., 2014).
Conformer generation: Conformer generation is an important step in 3D QSAR modeling. Herein, we have employed the Poling algorithm to generate maximum of 255 diverse conformations with energy threshold of 20 kcal/mol above the calculated energy minimum for every training set compounds. These conformation were predicted using the diverse conformation generation protocol, where Conformation method was set as FAST using the CHARMM input force field. In FAST conformation, conformational space of small molecules is generated using an efficient systematic search. If the molecule is too large, only one conformation is generated for each possible combination of stereocenters. Conformations for molecules that are neither too small nor too large, as measured by the flexibility of the molecule, are generated with a random search method that uses poling. In the conformation generation step, Maximum Systematic Conformations was set at 1000, Conformation Boltzmann was set at 300 and temperature cut-off was set to 0.2. Number of clusters was set to 20. The other parameter were kept default while conformation generation. This methodology was performed at the DS software v3.1 workspace (Mitra et al., 2010).
Training and test set preparation: Training set and test set compounds were generated for the eight dataset compounds along with the conformers. In quantitative activity relationship study, training set data is a set of compounds used to discover potentially predictive relationships. The test set is a set of data used in QSAR study to assess the strength and utility of a predictive relationship. Herein, Random splitting method of DS software was to generate the training and test set data. Training set percentage was set at 80 while generating the data set.
3D QSAR model generation and validation: QSAR modelling approach is used to generate predictive models correlating the biological activity with the structural descriptor of a molecule. In rational drug design methodology, QSAR plays pivotal role in the prediction of unknown compounds for their potency as optimized candidate lead (Dearden, 2003; Scholz et al., 2013). In 3D QSAR method, the energy potentials calculated using the 3D structures of a set of ligands are used as descriptors to build a model that relates the biological activities to the 3D structures. In most cases, these ligands all bind into the same binding site of the same or similar receptors. In the current investigation, 3D QSAR model was generated using the 8 dataset compounds including their conformers using DS software v3.1 (Dell Server in Windows). CHARMM force field is used and the electrostatic potential and the van der Waals potential are treated as separate terms. A +1e point charge is used as the electrostatic potential probe and distance-dependent dielectric constant is used to mimic the solvation effect. For the van der Waals potential a carbon atom with a 1.73 Angstrom radius is used as a probe. The energy grid potentials are filtered to remove highly correlated descriptors. A partial least square (PLS) model is then built using these remaining descriptors. Hence, the model was use to predict unknown compounds with malarial efficacy.
The partial least squares (PLS) model: Partial least squares regression is an extension of the multiple linear regression model. In PLS, rather than using all the independent variables (as in multiple linear regression), a small number of principal components is used. Partial least squares create a multiple-term linear equation based on a principal components analysis transformation of the independent variables. However, unlike a principal component analysis, the dependent variable is transformed as well. Axes are chosen that maximize retention of the variance and also correlate dependent and independent variables. More specifically, the covariance of a transformed independent variable with a transformed dependent variable is maximized.
As in multiple linear regression, the main purpose of partial least squares regression is to build a linear model: Y = X * B + E
Where, Y is a response matrix (or vector) formed by the dependent variables, X is a matrix formed by the independent variables, B is a matrix of the regression coefficients, and E is an error term for the model
Virtual screening (database searching): The generated model was applied to screen on 30,000 compounds from Chembridge chemical (Combiset) using DS software v3.1. The Chembridge (http://www.chembridge. com) library is a unique collection of small drug like compounds and useful for computer assisted screening (Wadood et al., 2014).
ADMET prediction: In silico ADMET study is an important step of CADD. Herein, ADMET properties of the screened ligands were studied and compared using the ADMET tool (module) of the Discovery Studio 3.1.
ADMET properties such as human intestinal absorption, aqueous solubility, blood brain barrier penetration, plasma protein binding, CYP2D6 binding and hepatotoxicity of the screens were predicted. TOPKAT module of toxicity prediction of DS was used to investigate the carcinogenicity and other toxicity of ligands.
Result and Discussion
Three dimensional quantitative activity relationship modeling is a most efficient and pivotal step in computer aided drug design. Eight dataset compounds including their conformers were considered in this study as presented in the Table I for model generation. Dataset was randomly splitted to training and test set data, where 626 compounds were found in training set and 157 in test set. Average conformations per ligand were computed as 97.9.
Table I: Training set compounds
Data set compounds | IC50 (µM) |
---|---|
4-Nerolidylcatechol | 0.7 |
4-Nerolidylcatechol SSD1 | 22.5 |
4-Nerolidylcatechol SSD2 | 3.9 |
4-Nerolidylcatechol SSD3 | 2.8 |
4-Nerolidylcatechol SSD4 | 0.7 |
4-Nerolidylcatechol SSD7 | 4.0 |
Catechol | 80.7 |
Safrole | 0.9 |
Development of regression models built from whole molecular steric and electrostatic fields can be useful for predicting activity and for visualizing favorable and unfavorable interactions. In this study, we have employed the structure of 4-nerolidylcatechol and their derivatives for generating a Partial Least-Squares (PLS) model is built using energy grids as descriptors.
The energy grids are computed using two probe types designed to measure electrostatic and steric effects. The validity of the model was obtained by leave-one-out (LOO), where internal predictive power was computed at 0.769 in terms of cross-validated correlation coefficient (q2). Component wise 5-fold cross validation result is presented in the Table II. Further the model was use to predict 30, 000 unknown chembridge compounds. Physiochemical properties calculation, ADME study and Toxicity profiling was performed for molecules fitted to the model.
Table II: 5-Fold cross validation result
Number of components | q2 | RMS error | Mean absolute error |
---|---|---|---|
1 | 0.467 | 1.867 | 1.238 |
2 | 0.536 | 1.786 | 1.192 |
3 | 0.556 | 1.774 | 1.145 |
4 | 0.561 | 1.768 | 1.146 |
5 | 0.561 | 1.766 | 1.140 |
6 | 0.560 | 1.767 | 1.140 |
Physiochemical properties of a compound or a drug are very much useful in discovery and development. Physiochemical properties of a ligand can be computed using its two dimensional structure. Herein, physicochemical properties computation was performed. We have predicted ~1500 compounds following lipinski's and Veber's drug likeness rule and fitted to the grid based QSAR model. Important properties such as number of rotatable bond, clogp, alogp etc with optimum score were computed.
ADMET investigation is a crucial step in drug discovery process. The Discovery Studio 3.1 was employed in ADME prediction study. Prebuilt validated model of ADMET models were used to compute the human intestinal absorption, Aqueous solubility (solubility of each compound in water at 25°C), blood brain barrier penetration, plasma protein binding CYP2D6 binding (cytochrome P450 2D6 enzyme inhibition) and hepatotoxicity (dose-dependent human hepatoxicity of compounds) of the screened compounds as presented in the Table III with favourable ADME characteristics. In this current study, we have identified seven compounds with good Solubility (-6.0<log (Sw) -2.0) level of 2-3 and moderate blood-brain barrier level. CYP2D6, hepatotoxic and plasma protein binding values of the screened compounds were found satisfactory.
Table III: ADME property prediction
SN | Compound ID | Solubility | Solubility level | Blood brain barrier | Blood brain barrier level | Cyp2D6 | Hepatoxic applicability | Plasma protein binding |
---|---|---|---|---|---|---|---|---|
1 | CD10097348 | -3.3 | 3 | -0.2 | 2 | -3.8 | 15.5 | -6.5 |
2 | CD10242133 | -3.0 | 3 | -0.6 | 3 | -5.4 | 15.0 | -6.1 |
3 | CD10374166 | -4.0 | 3 | -0.7 | 2 | -8.0 | 19.2 | 3.4 |
4 | CD11106591 | -3.7 | 3 | -0.3 | 2 | -1.5 | 16.4 | 1.2 |
5 | CD38160063 | -3.7 | 3 | -0.8 | 2 | -10.7 | 15.3 | 2.4 |
6 | CD75952907 | -3.4 | 3 | -0.5 | 2 | -6.1 | 16.9 | 3.0 |
7 | CD76675709 | -2.5 | 3 | -1.2 | 1 | -6.9 | 11.4 | -3.7 |
8 | CD96875226 | -2.9 | 3 | -0.6 | 3 | -3.3 | 12.9 | -2.3 |
9 | CD97711257 | -3.3 | 3 | -0.1 | 2 | -4.0 | 11.4 | -4.3 |
10 | CD97204986 | -3.8 | 3 | 0.1 | 1 | -3.9 | 15.8 | -2.0 |
11 | CD97550902 | -2.4 | 3 | -0.9 | 3 | -2.1 | 17.8 | -3.3 |
12 | CD97896374 | -3.5 | 3 | -0.6 | 3 | -2.7 | 15.2 | -3.8 |
13 | CD98749010 | -3.0 | 3 | -0.3 | 2 | -4.5 | 12.6 | -4.1 |
14 | CD98790017 | -3.0 | 3 | -0.3 | 2 | -3.9 | 13.2 | 0.3 |
15 | CD99939222 | -3.5 | 3 | -0.2 | 2 | -3.1 | 11.7 | -3.4 |
16 | CD10193496 | -4.4 | 2 | -0.9 | 3 | -14.4 | 15.7 | 0.0 |
17 | CD11219720 | -5.1 | 2 | -0.5 | 3 | -0.8 | 15.2 | -2.2 |
Toxicity profiling of a compound is a vital step in computational drug discovery process. Herein, the toxic and environmental effects of the screens were performed using the Toxicity Prediction by Komputer Assisted Technology (TOPKAT) module of Discovery Studio v3.1. TOPKAT uses robust and cross-validated Quantitative Structure Toxicity Relationship (QSTR) models for the computation of toxicity level of new compounds. We have predicted six non-carcinogenic compounds using the different TOPKAT modules as presented in the Table IV. These six compounds namely CD10097348, CD10374166, CD11106591, CD75952907, CD76675709 and CD96875226 (Figure 2) has also howed better in silico LD50 (g/kg body weight) and LC50 (mg/m3/h). Hence these lead compounds may be useful as a good starting point for the development of newer therapeutics of malaria with potential activity.
Figure 2: Screened non-toxic compounds; Compound ID, template base grid based model score; CD stand for chembridge database
Table IV: TOPKAT toxicity level
Compound ID | TOPKAT rat male NTP probability | TOPKAT rat male NTP enrichment | WOE probability | WOE enrichment | WOR score | Rat oral LD50 (g/kg body weight) |
Rat inhalational LC50 (mg/m3/h) |
---|---|---|---|---|---|---|---|
CD10097348 | 0.5 | 0.9 | 0.3 | 0.6 | -7.4 | 7.4 | 4.0 |
CD10374166 | 0.5 | 0.9 | 0.5 | 1.0 | -0.4 | 0.7 | 5.2 |
CD11106591 | 0.2 | 0.4 | 0.4 | 0.8 | -4.1 | 5.3 | 4.5 |
CD75952907 | 0.3 | 0.6 | 0.3 | 0.6 | -6.6 | 3.6 | 9.9 |
CD76675709 | 0.4 | 0.8 | 0.5 | 0.9 | -1.8 | 0.8 | 2.6 |
CD96875226 | 0.4 | 0.7 | 0.4 | 0.7 | -5.3 | 17.9 | 23.7 |
Conclusion
In summary, active antimalarial plant metabolite 4-nerolidylcatechol and their derivatives were employed to generate the PLP based 3D QSAR model. Predicted model identified six non-toxic database compounds namely CD10097348, CD10374166, CD11106591, CD75952907, CD76675709 and CD96875226 as a potential antimalarial lead.
Acknowledgements
The authors thankfully acknowledge the Department of Biotechnology, Government of India for providing the bioinformatics infrastructure facility to Dibrugarh University, Dibrugarh at Centre for Studies in Biotechnology and Prof. Alak Kr. Buragohain, Vice-Chancellor, Dibrugarh University for constant support and encouragement throughout the study.
References
Arooj M, Thangapandian S, John S, Hwang S, Park JK, Lee KW. 3D QSAR pharmacophore modeling, in silico screening, and density functional theory (DFT) approaches for identification of human chymase inhibitors, Int J Molec Sci. 2011: 12: 9236-64.
Bagatela BS, Lopes AP, Fonseca FL, Andreo MA, Nanayakkara DN, Bastos JK, Perazzo FF. Evaluation of antimicrobial and antimalarial activities of crude extract, fractions and 4-nerolidylcathecol from the aerial parts of Piper umbellata L. (Piperaceae). Nat Prod Res. 2013; 27: 2202-09.
Bharati K, Ganguly NK. Tackling the malaria problem in the South-East Asia Region: Need for a change in policy? Indian J Med Res. 2013; 137: 36-47.
Crompton PD, Moebius J, Portugal S, Waisberg M, Hart G, Garver LS, Miller LH, Barillas C, Pierce SK. Malaria immunity in man and mosquito: Insights into unsolved mysteries of a deadly infectious disease. Ann Rev Immunol. 2014; 32: 157-87.
Dearden JC. In silico prediction of drug toxicity. J Computer-aided Molec Design. 2003; 17: 119-27.
Ghosh A, Edwards MJ, Jacobs-Lorena M. The journey of the malaria parasite in the mosquito: Hopes for the new century. Parasitol Today. 2000; 16: 196-201.
Gogoi RR, Gogoi D, Bezbaruah RL. Virtual screening of compounds from Tabernaemontana divaricata for potential anti-bacterial activity. Bioinformation 2014; 10: 152-56.
Mitra I, Saha A, Roy K. Pharmacophore mapping of arylamino-substituted benzo[b]thiophenes as free radical scavengers. J Mol Model. 2010; 16: 1585-96.
Mohapatra PK, Prakash A, Bhattacharyya DR, Mahanta J. Malaria situation in north-eastern region of India, ICMR Bull. 1998; 28: 22-30.
NVBDCP. National vector borne diseases control programme. NVBDCP, Govt. of India, 2010. Available from: www.nvbdcp.gov.in
Pinto AC, Silva LF, Cavalcanti BC, Melo MR, Chaves FC, Lotufo LV, de Moraes MO, de Andrade-Neto VF, Tadei WP, Pessoa CO, Vieira PP, Pohlit AM. New antimalarial and cytotoxic 4-nerolidylcatechol derivatives. Eur J Med Chem. 2009; 44: 2731-35.
Rocha ESLF, Silva Pinto AC, Pohlit AM, Quignard EL, Vieira PP, Tadei WP, Chaves FC, Samonek JF, Lima CA, Costa MR, Alecrim M, Andrade-Neto VF. In vivo and in vitro antimalarial activity of 4-nerolidylcatechol. Phytotherapy Res. 2011; 25: 1181-88.
Scholz S, Sela E, Blaha L, Braunbeck T, Galay-Burgos M, Garcia-Franco M, Guinea J, Kluver N, Schirmer K, Tanneberger K, Tobor-Kaplon M, Witters H, Belanger S, Benfenati E, Creton S, Cronin MT, Eggen RI, Embry M, Ekman D, Gourmelon A, Halder M, Hardy B, Hartung T, Hubesch B, Jungmann D, Lampi MA, Lee L, Leonard M, Kuster E, Lillicrap A, Luckenbach T, Murk AJ, Navas JM, Peijnenburg W, Repetto G, Salinas E, Schuurmann G, Spielmann H, Tollefsen KE, Walter-Rohde S, Whale G, Wheeler JR, Winter MJ. A European perspective on alternatives to animal testing for environmental hazard identification and risk assessment. Regulatory Toxicol Pharmacol. 2013; 67: 506-30.
Lima ES, Pinto AC, Nogueira KL, Almeida PD, Vasconcellos MC, Chaves FC, Tadei WP, Pohlit AM. Stability and antioxidant activity of semi-synthetic derivatives of 4-nerolidylcatechol. Molecules 2012; 18: 178-89.
Wadood A, Riaz M, Uddin R, Ul-Haq Z. In silico identification and evaluation of leads for the simultaneous inhibition of protease and helicase activities of HCV NS3/4A protease using complex based pharmacophore mapping and virtual screening. PloS one 2014; 9: e89109.
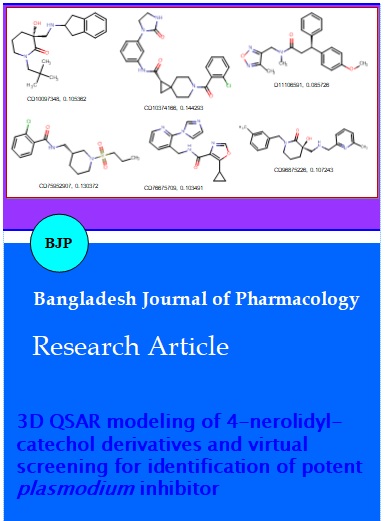