Hexonic derivatives as human GABA-AT inhibitors: A molecular docking approach
Abstract
Human gamma-aminobutyric acid aminotransferase (GABA-AT), a pyridoxal phosphate dependent enzyme is responsible for the degradation of the inhibitory neurotransmitter GABA. Currently, GABA-AT is a potential drug target for epilepsy due to the selective inhibition in brain. In this computational study, we mainly focus on screening of novel lead candidates against GABA-AT using hexonic derivatives. Structure based virtual screening is performed in Vina that screened top hits based on least binding affinity. Further re-docking on hits is performed in AutoDock results in identification of leads with favorable binding energy and hydrogen bond interactions confirmed the effective inhibition. In conclusion, leads 3-aminohex-5-enoic acid and AG-E-60842 can acts as specific leads for GABA-AT and assist in discovery of novel antiepileptic drugs.
Introduction
In mammalian central nervous system, gamma-aminobutyric acid (GABA) is a predominant inhibitory neurotransmitter that involved in modulation of central inhibitory tone via activation of various receptors like GABAA, GABAC (Osolodkin et al., 2009; Smith and Simpson, 2003). Convulsions observed in neurological disorder are due to depleted levels of GABA in brain (Karlsson et al., 1974). Enzyme present in the brain is γ-aminobutyric acid aminotransferase (GABA-AT) involved in regulation of GABA level in brain. Studies on GABA showed that it is not an effective anticonvulsive agent as it does not cross the blood brain barrier which related to process of xenobiotics entering the brain (Silverman et al., 1986). With the effect of GABA-AT inhibition, increase of GABA concentration in brain thus acts as drug target for anti-epilepsy drugs (Clift and Silverman, 2008). Current antiepileptic drugs belong to class of oxcarbazepine, gabapentin, levetiracetam, lamotrigine and topiramate that possess with diverse mechanisms of action. Targeting GABA-AT for the specific inhibition can be a better mechanism for identification of novel antiepileptic drugs.
Materials and Methods
Structural modelling of human GABA-AT: In order to carry out the computational inhibitor design, three dimensional structure of human GABA-AT was needed. Due to the absence of experimental structure and no public available structural model, we performed structural modeling using homology modeling method. The query protein sequence of GABA-AT was retrieved from Uniprot database with accession No: P80404. The suitable template was identified using NCBI-BLAST (Altschul et al., 1990) with homolog family, high percentage of sequence similarity and high score. Using the identified template, the structural model was generated by Modeller version 9v11 software (Eswar et al., 2006). Modeller was mainly based on algorithm with satisfaction of conformational restraints. With the use of align2d.py" and "model-default.py" programs, structural model was generated from template coordinates and followed by energy minimization done by molecular dynamics procedures results in "mol pdf" values (molecular probability density function). From the top three models, best model was selected from one with least mol.pdf value. The structural evaluation of GABA AT model was done using Ramachandran plot construction in Procheck server (Laskowski et al., 1993). Finally, the visualization of the structural model was done by using Rasmol software (Sayle and Milner-White, 1995).
Active site prediction: After predicting the structure of human GABA-AT, the possible active binding sites of GABA-AT were predicted using Q-SiteFinder tool (Laurie and Jackson, 2005). The tool used the interaction energy between the query protein structure and van der Waals probe for the identification of binding sites. Ten binding sites were obtained for query protein and the best site was selected based on ranking with sum of interactions energies for sites within clusters.
Hit identification by virtual screening: For hit identification, structure-based virtual screening was performed in Vina software (Trott, Olson 2010) with hexonic derivatives targeting active sites of GABA-AT. The initial step in virtual screening was preparation of query protein and ligands files in PDBQT format. The next step was preparation of configuration file with volume of the grid box fixed to 27000 Angstrom, centre x= 0.15, y= 3.56 and z= 2.64, size 60 x 60 x 60 Angstrom was used. The critical search algorithm with exhaustiveness parameter set to 8 for accuracy. Vina software evaluated the docking results by binding affinity in kcal/mol. Top hits from the docking results were selected based on the least binding affinity among the dataset.
Lead identification by molecular docking analysis: For lead identification, molecular docking on hits was performed using AutoDock 4.2 software (Morris et al., 1998). The basic steps of docking were preparation of protein and ligand. The program AutoTors was used in preparation of ligand with defined root and flexible nature to ligand. After adding Gasteiger charges to the ligands, the ligand molecule saved in PDBQT format. The protein molecular was prepared by adding Kollman charges, removing water molecule and saved in PDBQT format. The grid size was set to 60 x 60 x 60 points with grid spacing of 0.375 Angstrom for active site. The search algorithm was used in AutoGrid program based on defined active sites active and generated the grid parameter files. Followed by scoring algorithm in Autodock program was used for binding conformation of ligand. Using Lamarckian genetic algorithm (LGA), ten runs of docking were performed with maximum number of 2,500,000 energy evaluations of 150 individuals was generated for the docking runs. The post docking analysis evaluated with least binding energy for the selection of best binding conformation of GABA-AT/ligand complex. The hydrogen bond interactions were analyzed by PyMOL software (DeLano, 2009).
Result and Discussion
Though structural model of human GABA-AT was reported (Khan et al., 2011), but the structure is not public available for further analysis. Thus, we carried out homology modeling of human GABA-AT for in-built structure for inhibitor design. The query protein GABA-AT contains 500 amino acids and showed more sequence identity to 4-Aminobutyrate-aminotransferase from pig PDB ID: 1OHV (Storici et al., 2004). With 1OHV as template, the three dimensional model of GABA-AT was generated by using Modeller 9v11. The software used the algorithm with satisfaction of spatial restraints from template in order to generate model. Among the top three models, the best model with least mol.pdf value of 2442.502 was selected. GABA-AT model showed the structure contains more helices and less beta sheet and fall under the structural classification of alpha/beta proteins. The structural evaluation of model was performed by Ramachandran plot in PRO-CHECK server. The built model contains core region of 91.8%, additional allowed region of 7.2 % and 0.8 of generously allowed and no disallowed region. Thus, the protein model was structurally significant with proper conformation contains acceptable range of rotational angles like phi/psi (Agarwal et al., 2013). The human GABA-AT model was visualized in Rasmol with the secondary structure elements shown in Figure 1.
Figure 1: In built structural model of human GABA-AT generated from Modeller 9V11. Rasmol was used for the structure visualization represented the helices, strands, loop and turns
After structural modeling, the critical step in inhibitor design was prediction of active binding pocket and binding sites. Using Q-SiteFinder, the active binding pocket was predicted based on highest ranking and guide to protein-ligand docking (Rohini and Srikumar, 2013) shown in Figure 2. The deep cavity of active binding pocket was the target region for GABA-AT inhibition. The predicted active site residues include Ala32, Val33, His34, Phe35, Phe36, Cys37, Tyr39, Leu47, Asp57, Tyr59, Ser60, Gln61, Ile62 and Tyr69. The sites were more sequential in order to cover the cavity region in GABA-AT. The grid box was constructed based on binding pocket and large enough for the search space used for docking analysis.
Figure 2: Active binding pocket was represented in green color obtained from Q-SiteFinder
The rational inhibitor design GABA-AT using class of hexonic acid derivatives as ligand dataset shown in Figure 3. The ligand properties of hexonic derivaties were listed in Table I and all ligands satisfied the Lipinski's rule of five. Molecular weight of the ligands was in range of 490 to 129 g/mol. Also, the hydrogen bond donor and acceptor were in range for the favorable binding with the target protein. Structure based virtual screening was applied for structural model against hexonic derivatives for hit identification and drug discovery (Cheng at al., 2012). AutoDock Vina was more accurate in screening based on stochastic global optimization and better search algorithm (Rajesh et al., 2014). After successful virtual screening in AutoDock Vina, the hits were identified based on binding affinity from the ten runs for each derivative (Yadav and Singh, 2013). The top ligands 3-aminohex-5-enoic acid and AG-E-60842 evaluated by least binding affinity more than -7 Kcal/mol confirmed the effective binding and selected as hits shown in Table II. The moderate binding was observed in remaining ligands with binding energy in range of -4 to -6 kcal/mol. The top hits were selected for further docking for the confirmation of binding pose and hydrogen bond interactions.
Table I: Ligand properties
SL. No. | Ligand Name | Compound ID | Mol.Wt g/mol |
XLogP3-AA | Donor HB | Acceptor HB |
---|---|---|---|---|---|---|
1 | 3-Deoxy arabino-hexonic acid | 152990 | 180.1 | -2.4 | 5 | 6 |
2 | D-Arabino-hexonic acid | 188315 | 180.1 | -2.8 | 5 | 6 |
3 | 2-Deoxy-D-lyxo-hexonic acid | 152195 | 180.1 | -2.8 | 5 | 6 |
4 | 2-Deoxyhexonic acid | 626 | 180.1 | -2.8 | 5 | 6 |
5 | Tetrahydrocannabinol C4'-glucuronide | 194258 | 490.6 | 3.5 | 5 | 8 |
6 | 6-o-[n,n-Di(propan-2-yl)glycyl]hexonic acid | 9356 | 337.4 | -3.4 | 5 | 9 |
7 | 3-Deoxy-d-lyxo-hexonic acid | 5289313 | 180.1 | -2.4 | 5 | 6 |
8 | (4R-Cis)-6-Hydroxymethyl-2,2-dimethyl-1,3-dioxane-4-acetic acid 1,1-dimethylethyl ester | 9816650 | 260.3 | 1 | 1 | 5 |
9 | (2S,5S)-2,6-Diamino-5-hydroxyhexanoic acid | 439437 | 162.2 | -4.2 | 4 | 5 |
10 | AG-E-60842 | 46737716 | 301.4 | 2.3 | 1 | 5 |
11 | Tert-butyl[(4R,6R)-6-(2-aminoethyl)-2,2-dimethyl-1, 3-dioxan-4-yl]acetate | 29922444 | 259.3 | 0.8 | 1 | 5 |
12 | 3-Aminohex-5-enoic acid | 11367001 | 129.1 | -1.9 | 2 | 3 |
Table II: Virtual screening results from Vina
SL. No. | Ligand name | Binding affinity (Kcal\mol) |
---|---|---|
1 | 3-Deoxy arabino-hexonic acid | -4.8 |
2 | D-Arabino-hexonic acid | -4.2 |
3 | 2-Deoxy-D-lyxo-hexonic acid | -5.1 |
4 | 2-Deoxyhexonic acid | -5.6 |
5 | Tetrahydrocannabinol C4'-glucuronide | -6.2 |
6 | 6-o-[n,n-Di(propan-2-yl)glycyl]hexonic acid | -6.5 |
7 | 3-Deoxy-d-lyxo-hexonic acid | -5.4 |
8 | (4R-Cis)-6-Hydroxymethyl-2,2-dimethyl-1,3-dioxane-4-acetic acid 1,1-dimethylethyl ester | -6.4 |
9 | (2S,5S)-2,6-Diamino-5-hydroxyhexanoic acid | -4.6 |
10 | AG-E-60842 | -7.6 |
11 | Tert-butyl[(4R,6R)-6-(2-aminoethyl)-2,2-dimethyl-1, 3-dioxan-4-yl]acetate | -6.2 |
12 | 3-Aminohex-5-enoic acid | -7.8 |
Figure 3: The chemical structure of hexonic derivatives used in the study
The re-docking on screened hits was performed in molecular docking analysis for leads identification with the possible binding mode, least binding energy and hydrogen bond interactions between GABA-AT/ligand complexes. Molecular docking, a method predict the preferred orientation of one molecule to a second when bound to each other to form stable complex (Rangaraju and Rao, 2013). Hence, molecular docking plays an important role in the rational design of inhibitors against drug targets. Hydrogen bond, a non-covalent interaction occurs between protein and ligand described as more critical in protein inhibition towards inhibitor design (Panigrahi and Desiraju, 2007). From the docking results, top 10 poses with least binding energy was selected as best conformation of the two ligand complexes. The hit1 3-aminohex-5-enoic acid showed the binding pose of ligand with GABA-AT active binding pocket. The binding energy of hit1 was -6.8 kcal/mol and with four hydrogen bond interactions observed in PyMOL shown in Figure 4a. The hit2 AG-E-60842 showed the binding pose of ligand with GABA-AT active binding pocket. The binding energy of hit2 was -6.6 kcal/mol and with three hydrogen bond interactions observed in PyMOL shown in Figure 4b. The critical residues ASP 57, TYR 59 and SER 60 of GABA-AT were involved in hydrogen bond interactions with proper bond length shown in Table III.
Table III: Hydrogen bond interactions analysis from AutoDock results
Sl. No. | Ligand name | Ligand atom | Protein atom | Bond length (Angstrom) |
---|---|---|---|---|
1 | 3-Aminohex-5-enoic acid | H H H O |
OE1 (TYR 59) N (SER 60) OD1 (SER 60) NH (ASP 57) |
2.7 2.2 2.4 3.0 |
2 | AG-E-60842 | N H N |
OG1(ASP 57) OG1 (SER 60) OD1 (SER 60) |
2.8 2.4 2.2 |
Figure 4: Binding pose of GABA-AT/ligand complexes (a) 3-aminohex-5-enoic acid (b) AG-E-60842 obtained from AutoDock result. The visualization was done by PyMOL and hydrogen bond interactions were showed in yellow color
In discussion, the structural model of human GABA-AT was more significantly modeled by homology modeling and used for the computational inhibitor study. The critical active sites and binding pocket was predicted well using Q-SiteFinder. Hexonic derivatives followed the Lipinski's rule of five and confirmed the druglikeness of ligands. Virtual screening was more useful in screening the top two hits from the ligand dataset based on least binding affinity in AutoDock Vina. Subsequent re-docking on hits in AutoDock identified the binding mechanism of GABA-AT inhibition through hydrogen bond interactions. Least binding energy of hits also supported the role of energy during complex formation. Overall, both 3-aminohex-5-enoic acid and AG-E-60842 acts as novel lead candidates for human GABA-AT.
Conclusion
The computational inhibitor study on human GABA-AT confirmed the active inhibition by hexonic derivatives. The reported leads 3-aminohex-5-enoic acid and AG-E-60842 as indispensible GABA-AT inhibitors and in future can acts as novel antiepilepsy drugs with further experimental studies.
Acknowledgement
The authors thank the management of VIT University for providing the facilities to carry out this work.
References
Agrawal P, Thakur Z, Kulharia M. Homology modeling and structural validation of tissue factor pathway inhibitor. Bioinformation. 2013; 9: 808-12.
Altschul SF, Gish W, Miller W, Myers EW, Lipman DJ. Basic local alignment search tool. J Mol Biol. 1990; 215: 403-10.
Bolton E, Wang Y, Thiessen PA, Bryant SH. PubChem: Integrated platform of small molecules and biological activities. In: Annual reports in computational chemistry. Vol 4. 2008.
Cheng T, Li Q, Zhou Z, Wang Y, Bryant SH. Structure-based virtual screening for drug discovery: A problem-centric review. AAPS J. 2012; 14: 133–41.
Clift MD, Silverman RB. Synthesis and evaluation of novel aromatic substrates and competitive inhibitors of GABA aminotransferase. Bioorg Med Chem Lett. 2008; 18: 3122–25.
DeLano WL. Pymol. Portland, OR, Schrodinger LLC, 2009.
Eswar N, Marti-Renom MA, Webb B, Madhusudhan MS, Eramian D, Shen M, Pieper U, Sali A. Comparative protein structure modeling with MODELLER. Curr Protoc Bioinformatics. Chapter 5. Unit 5.6. 2006.
Karlsson A, Fonnum F, Malthe-Sorenssen D, Storm-Mathisen J. Effect of the convulsive agent 3-mercaptopropionic acid on the basal levels of GABA, other amino acids and glutamate decarboxylase in different regions of the rat brain. Biochem. Pharmacol. 1974; 23: 3053-61.
Khan HN, Rashid H, Kulsoom S. Homology modeling of γ-aminobutyrate-aminotransferase, a pyridoxal phosphate-dependent enzyme of Homo sapiens: Molecular modeling approach to rational drug design against epilepsy. Afr J Biotechnol. 2011; 10: 5916-26.
Laskowski RA, MacArthur MW, Moss DS, Thornton JM. PROCHECK: A program to check the stereochemical quality of protein structures. J App Cryst. 1993; 26: 283-91.
Laurie ATR, Jackson RM. Q-SiteFinder: An energy-based method or the prediction of protein–ligand binding sites. Bioinformatics. 2005; 21: 1908-16.
Morris GM, Goodsell DS, Halliday RS, Huey R, Hart WE, Belew RK, Olson AJJ. Automated docking using a Lamarckian genetic algorithm and an empirical binding free energy function. J Comput Chem. 1998; 19: 1639-62.
Osolodkin DI, Chupakhin VI, Palyulin VA, Zefirov NS. Molecular modeling of ligand-receptor interactions in GABA C receptor. J Mol Graph Model. 2009; 27: 813-21.
Panigrahi SK, Desiraju GR. Strong and weak hydrogen bonds in the protein-ligand interface. Proteins 2007; 67: 128-41.
Rangaraju A, Rao AV. A review on molecular docking: Novel tool in drug design and analysis. J Harmon Res Pharm. 2013; 2: 215-21.
Rajesh PK, Rohini K, Srikumar PS. Anti-tuberculosis drugs against mycobacterium tuberculosis PKNB and mutants I33D/ D76A: A comparative docking study. Int J Pharm Pharm Sci. 2014; 6: 662-64.
Rohini K, Srikumar PS. Structural insights on mycobacterium tuberculosis thiazole synthase: A molecular dynamics/docking approach. Appl Biochem Biotechnol. 2013; 169: 1790-98.
Sayle RA, Milner-White EJ. RasMol: Biomolecular graphics for all. Trends Biochem Sci. 1995; 20: 374.
Silverman RB, Durkee SC, Invergo BJ. 4-Amino-2-(substituted methyl)-2-butenoic acids: Substrates and potent inhibitors of γ-aminobutyric acid aminotransferase. J Med Chem. 1986; 29: 764-70.
Smith AJ, Simpson PB. Methodological approaches for the study of GABAA receptor pharmacology and functional responses. Anal Bioanal Chem. 2003; 377: 843–51.
Storici P, De Biase D, Bossa F, Bruno S, Mozzarelli A, Peneff C, Silverman RB, Schirmer T. Structures of gamma-aminobutyric acid (GABA) aminotransferase, a pyridoxal 5'-phosphate, and [2Fe-2S] cluster-containing enzyme, complexed with gamma-ethynyl-GABA and with the antiepilepsy drug vigabatrin. J Biol Chem. 2004; 279: 363-73.
Tetko IV, Gasteiger J, Todeschini R, Mauri A, Livingstone D, Ertl P, Palyulin VA, Radchenko EV, Zefirov NS, Makarenko AS, Tanchuk VY, Prokopenko VV. Virtual computational chemistry laboratory design and description. J Comput Aid Mol Des. 2005; 19: 453-63.
Trott O, Olson AJ. AutoDock Vina: Improving the speed and accuracy of docking with a new scoring function, efficient optimization, and multithreading. J Comput Chem. 2010; 31: 455–61.
Yadav M, Singh G. Virtual screening of ligand molecules for target protein CYP26A1 by using AutoDock-Vina. Int J Innov Res Sci Eng Technol. 2013; 2: 4915-20.
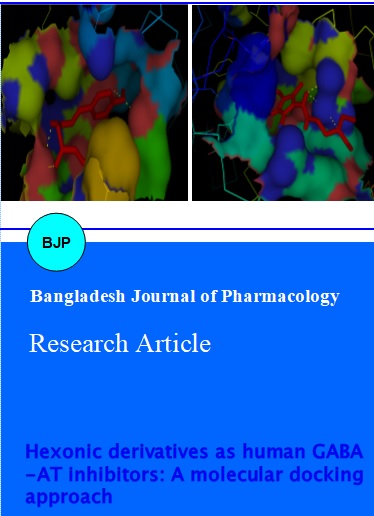