Discovery of potential cyclooxygenase inhibitors using in silico docking studies
Abstract
This study deals with the evaluation of the cyclooxygenase inhibitory activity of flavonoids (tangeritin, morin, rhamnetin, theaflavin, pachypodol, eriodictyol, homoeriodictyol, aromadedrin, okanin) using in silico docking studies. In silico docking studies were carried out using AutoDock 4.2. Three important parameters like binding energy, inhibition constant and intermolecular energy were determined. The results showed that all the selected flavonoids showed binding energy ranging between -8.8 kcalmol-1 to -6.1 kcal mol-1 when compared with that of the standard (-8.3 kcalmol-1). Intermolecular energy (-10.6 kcalmol-1 to -7.9 kcalmol-1) and inhibition constant (373.9 nM to 34.0 µM) of the ligands also coincide with the binding energy. All the selected flavonoids contributed cyclooxygenase inhibitory activity because of its structural parameters. These molecular docking analyses could lead to the further development of potent cyclooxygenase inhibitors.
Introduction
Drug design is an important tool in the field of medicinal chemistry where new compounds are synthesized by molecular or chemical manipulation of the lead moiety in order to produce highly active compounds with minimum steric effect (Cavasotto and Abagyan, 2004). Nowadays, the use of computers to predict the binding of libraries of small molecules to known target structures is an increasingly important component in the drug discovery process (Shoichet, 2004; Koppen, 2009). There is a wide range of software packages available for the conduct of molecular docking simulations like, AutoDock, GOLD, FlexX (Collignon et al., 2011).
AutoDock 4.2 is the most recent version which has been widely used for virtual screening, due to its enhanced docking speed (Schames et al., 2004). Its default search function is based on Lamarckian Genetic Algorithm (LGA), a hybrid genetic algorithm with local optimization that uses a parameterized free-energy scoring function to estimate the binding energy. Each docking is comprised of multiple independent executions of LGA and a potential way to increase its performance is to parallelize the aspects for execution (Cosconati et al., 2010). Docking of small molecules in the receptor binding site and estimation of binding affinity of the complex is a vital part of structure based drug design (Seeliger and Groot, 2010).
Inflammation is a process involved in the pathogenesis of several disorders like arthritis and cardiovascular disease (Jiang and Ames, 2003). Cyclooxygenase (COX) is an endogenous enzyme which catalyses the conversion of arachidonic acid into prostaglandins and thromboxanes (Smith et al., 2000; Vane et al., 1998). The enzyme exists in at least two isoforms, COX-1 and COX-2. Although both the isoforms catalyze the same biochemical transformation, the two isoforms are subject to a different expression regulation (Smith et al., 1993). COX-1 is a constitutive enzyme and is responsible for the supply of prostaglandins which maintain the integrity of the gastric mucosa and provide adequate vascular homeostasis whereas COX-2 is an inducible enzyme and is expressed only after an inflammatory stimulus (Kurumbail et al., 1996; Herschman, 1996).
Flavonoids belong to a group of natural substances with variable phenolic structures and are found in fruit, vegetables, grains, bark, roots, stems, flowers, tea, and wine (Middleton, 1998). These natural products were known for their beneficial effects on health long before flavonoids were isolated as the effective compounds. More than 4,000 varieties of flavonoids have been identified, many of which are responsible for the attractive colors of flowers, fruit, and leaves (Groot and Rauen, 1998). Research on flavonoids received an added impulse with the discovery of the French paradox, the low cardiovascular mortality rate observed in Mediterranean populations in association with red wine consumption and a high saturated fat intake. The flavonoids in red wine are responsible, at least in part, for this effect (Formica and Regelson, 1995).
However there is no conclusive report as to whether the anti-inflammatory activity of the flavonoids. The stereochemistry of binding of the flavonoids on cyclooxygenase has not yet been characterized. In the present study, the structural models of the ligands in the cyclooxygenase binding sites has been carried out, which may facilitate further development of more potent anti-inflammatory agents.
Materials and Methods
Software required
Python 2.7 - language was downloaded from www.python.com, Cygwin (a data storage) c:\program and Python 2.5 were simultaneously downloaded from www.cygwin.com, Molecular graphics laboratory (MGL) tools and AutoDock4.2 was downloaded from www.scripps.edu, Discovery studio visualizer 2.5.5 was downloaded from www.accelerys.com, Molecular orbital package (MOPAC), Chemsketch was downloaded from www.acdlabs.com. Online smiles translation was carried out using cactus.nci.nih.gov/translate/.
Docking methodology
We employed the Lamarckian genetic algorithm (LGA) for ligand conformational searching, which is a hybrid of a genetic algorithm and a local search algorithm. This algorithm first builds a population of individuals (genes), each being a different random conformation of the docked molecule. Each individual is then mutated to acquire a slightly different translation and rotation and the local search algorithm then performs energy minimizations on a user-specified proportion of the population of individuals. The individuals with the low resulting energy are transferred to the next generation and the process is then repeated. The algorithm is called Lamarckian because every new generation of individuals is allowed to inherit the local search adaptations of their parents.
An extended PDB format, termed as PDBQT file was used for coordinate files which includes atomic partial charges. AutoDock Tools was used for creating PDBQT files from traditional PDB files (Khodade et al., 2007). Crystal structure of cyclooxygenase enzyme was downloaded from the Brookhaeven protein data bank (Figure 1).
Figure 1: Cyclooxygenase enzyme from RCSB (4COX)
In Figure 2, the flavonoid ligands like tangeritin, morin, rhamnetin, theaflavin, pachypodol, eriodictyol, homoeriodictyol, aromadedrin, okanin and celecoxib were built using Chemsketch and optimized using "Prepare Ligands" in the AutoDock 4.2 for docking studies. The optimized ligand molecules were docked into refined cyclooxygenase model using "LigandFit" in the AutoDock 4.2 (Goodsell et al., 1996).
Figure 2: The optimized ligand molecules (1 Tangeritin, 2 Morin, 3 Rhamnetin, 4 Theaflavin, 5 Pachypodol, 6 Eriodictyol, 7 Homoeriodictyol, 8 Aromadedrin, 9 Okanin and 10 Celecoxib)
The preparation of the target protein 4COX (unbound target) with the AutoDock Tools software involved adding all hydrogen atoms to the macromolecule, which is a step necessary for correct calculation of partial atomic charges. Gasteiger charges are calculated for each atom of the macromolecule in AutoDock 4.2 instead of Kollman charges which were used in the previous versions of this program. Three-dimensional affinity grids of size 277 x 277 x 277 Å with 0.6 Å spacing were centered on the geometric center of the target protein and were calculated for each of the following atom types: HD, C, A, N, OA, and SA, representing all possible atom types in a protein. Additionally, an electrostatic map and a desolvation map were also calculated (Konc et al., 2011).
Rapid energy evaluation was achieved by precalculating atomic affinity potentials for each atom in the ligand molecule. In the AutoGrid procedure, the target enzyme was embedded on a three dimensional grid point (Morris et al., 2007). The energy of interaction of each atom in the ligand was encountered.
We have selected important docking parameters for the LGA as follows: Population size of 150 individuals, 2.5 million energy evaluations, maximum of 27000 generations, number of top individuals to automatically survive to next generation of 1, mutation rate of 0.02, crossover rate of 0.8, 10 docking runs, and random initial positions and conformations. The probability of performing local search on an individual in the population was set to 0.06. Unbound target 4COX and unbound ligands were both treated as rigid.
AutoDock was run several times to get various docked conformations, and used to analyze the predicted docking energy. The binding sites for these molecules were selected based on the ligand-binding pocket of the templates (Chang et al., 2010). AutoDock Tools provide various methods to analyze the results of docking simulations such as, conformational similarity, visualizing the binding site and its energy and other parameters like intermolecular energy and inhibition constant. For each ligand, ten best poses were generated and scored using AutoDock 4.2 scoring functions (Park et al., 2006).
Result and Discussion
The docking poses were ranked according to their docking scores and both the ranked list of docked ligands and their corresponding binding poses (Madeswaran et al., 2011). In Figure 3, docked pose of cyclooxygenase enzyme with the ligands Rhamnetin and Celecoxib clearly demonstrated the binding positions of the ligand with the enzyme. Binding energy of the individual compounds were calculated using the following formula,
Binding energy = A + B + C - D
where, A denotes final intermolecular energy + Wandervalls energy (vdW) + hydrogen bonds + desolvation energy + electrostatic energy (kcalmol-1), B denotes final total internal energy (kcalmol-1), C denotes torsional free energy (kcalmol-1), D denotes unbound system's energy (kcalmol-1).
In Figure 3, the binding sites of the most active flavonoid Rhamnetin was cleary showed and it was found to be, Phe 357, Leu 359, Tyr 355, Gly 354, Val 349, Ile 345, Val 116, Val 344, Leu 534, Leu 531, Ser 530, Ala 527, Phe 205, Gly 526, Tyr 385, Phe 381, Tyr 387, Ser 353.
Figure 3: Docked pose of cyclooxygenase enzyme (4COX) with rhamnetin
In Figure 4, the potential binding sites of the standard was clearly showed and it was found that, Met 535, Leu 534, Lys 532, Leu 117, Met 113, Leu 531, Ser 530, Val 349, Ala 527, Gly 526, Phe 381, Tyr 385,Trp 387, Met 522, Val 523, Leu 352 and Ser 353. This proves that the effective binding sites are present in the selected flavonoid rhamnetin when compared with the celecoxib. It proves that the ability of inhibiting the aldose reductase enzyme by the selected flavonoid.
Figure 4: Docked pose of cyclooxygenase enzyme (4COX) with celecoxib
Analysis of the receptor/ligand complex models generated after successful docking of the flavonoids was based on the parameters such as hydrogen bond interactions, II--II interactions, binding energy, RMSD of active site residues and orientation of the docked compound within the active site (Umamaheswari et al., 2011). As a general rule, in most of the potent anti inflammatory compounds, both hydrogen bond and II-II hydrophobic interactions between the compound and the active sites of the receptor have been found to be responsible for mediating the biological activity.
Flavonoids showed binding energy ranging between -8.8 kcalmol-1 to -6.1 kcalmol-1 (Table I). All the selected flavonoids had showed binding energy compared to that of celecoxib (-8.3 kcalmol-1). This proves that flavonoids consist of potential cyclooxygenase inhibitory binding sites similar to that of the standard.
In addition, two other parameters like inhibition constant (Ki) and intermolecular energy were also determined. Flavonoids showed inhibition constant ranging from 373.9 nM to 34.0 µM (Table II). All the selected compounds had lesser inhibition constant when compared to the standard (826.1 nM). Inhibition constant is directly proportional to binding energy. Thus, the cyclooxygenase inhibitory activity of the flavonoids were compared with the celecoxib.
As shown in Table III, flavonoids showed intermolecular energy ranging between -10.6 kcalmol-1 to-7.9 kcalmol-1 which was lesser when compared to the standard (-9.8 kcalmol-1). Intermolecular energy is also directly proportional to binding energy. We found a decrease in intermolecular energy of all the selected compounds with a simultaneous decrease in the binding energy. This result further proved the cyclooxygenase inhibitory activity of all the selected flavonoids.
Based on the docking studies, the cyclooxygenase inhibitory activity of the selected compounds was found to be decreased in the order of rhamnetin, tangeritin, celecoxib, eriodictyol, homoeriodictyol, aromadedrin, okanin, morin, theaflavin and pachypodol. On the basis of the above study, rhamnetin, tangeritin, eriodictyol, homoeriodictyol and aromadedrin possess potential cyclooxygenase inhibitory binding sites similar to that of the standard. This may be attributed due to the differences in the position of the functional groups in the compounds.
Conclusion
Flavonoids especially, rhamnetin, tangeritin, eriodictyol, homoeriodictyol and aromadedrin have excellent binding interactions with cyclooxygenase.
References
Cavasotto CN, Abagyan RA. Protein flexibility in ligand docking and virtual screening to protein kinases. J. Mol. Biol. 2004; 12: 209-25.
Chang MW, Ayeni C, Breuer S. Virtual screening for HIV protease inhibitors: A comparison of AutoDock 4 and vina. PLoS ONE. PLoS ONE. 2010; 5: 119-55.
Collignon B, Schulz R, Smith JC. Task-parallel message passing interface implementation of AutoDock4 for docking of very large databases of compounds using high-performance supercomputers. J Comput Chem. 2011; 32: 1202-09.
Cosconati S, Forli S, Perryman AL, Harris R, Goodsell DS, Olson AJ. Virtual Screening with AutoDock: Theory and practice. Exp Opin Drug Disc. 2010; 5: 597-607.
Formica JV, Regelson W. Review of the biology of quercetin and related bioflavonoids. Food Chem Toxicol. 1995; 33: 1061-80.
Goodsell DS, Morris GM, Olson AJ. Automated docking of flexible ligands: Applications of AutoDock. J Mol Recog. 1996; 9: 1-5.
Groot H, Rauen U. Tissue injury by reactive oxygen species and the protective effects of flavonoids. Fundam Clin Pharmacol. 1998; 12: 249-55.
Herschman HR. Prostaglandin synthase 2. Biochim Biophys Acta Lipids Lipid Metab. 1996; 1299: 125-40.
Jiang Q, Ames BN. λ-Tocopherol, but not α-tocopherol, decreases proinflammatory eicosanoids and inflammation damage in rats. FASEB. 2003; 17: 816-22.
Khodade P, Prabhu R, Chandra N. Parallel implementation of Autodock. J App Crystal. 2007; 40: 598-99.
Konc J, Konc JT, Penca M, Janezic M. Binding-sites prediction assisting protein-protein docking. Acta Chim Slov. 2011; 58: 396-401.
Koppen H. Virtual screening: What does it give us? Curr Opin Drug Disc Dev. 2009; 12: 397-407 .
Kurumbail RG, Stevens AM, Gierse JK, McDonald JJ, Stegeman RA, Park JY, Gildehaus D, Miyashiro JM, Penning TD, Seibert K, Isakson PC, Stallings WC. Structural basis for selective inhibition of cyclooxygenase 2 by antiinflammatory agents. Nature 1996; 384: 644.
Madeswaran A, Umamaheswari M, Asokkumar K, Sivashanmugam T, Subhadradevi V, Jagannath P. Docking studies: In silico lipoxygenase inhibitory activity of some commercially available flavonoids. Bangladesh J Pharmacol. 2011; 6: 117-23.
Middleton EJ, Effect of plant flavonoids on immune and inflammatory cell function. Adv Exp Med Biol. 1998; 439: 175-82.
Morris GM, Huey R, Lindstrom W, Sanner MF, Belew RK, Goodsell DS, Olson JA. J Comput Chem. 2009; 30: 2785-91.
Park H, Lee J, Lee S. Critical assessment of the automated AutoDock as a new docking tool for virtual screening. Proteins 2006; 65: 549-54.
Schames JR, Henchman RH, Seigel JS, Sotriffer CA, Ni H, McCammon A. Discovery of a Novel Binding Trench in HIV Integrase. J Med Chem. 2004; 47: 1879-81.
Schoichet BK. Virtual screening of chemical libraries. Nature 2004; 43: 862-65.
Seeliger D, Groot BL. Ligand docking and binding site analysis with PyMOL and AutoDock/Vina. J Comput Aided Mol Des. 2010; 24: 417-24.
Smith CJ, Morrow JD, Roberts LJI, Marnett LJ. Differentiation of monocytoid THP-1 cells with phorbol ester induces expression of prostaglandin endoperoxide synthase-1 (COX-1). Biochem Biophys Res Commun. 1993; 192: 787-93.
Smith RM, DeWitt DL, Garavito RM. Cyclooxygenases: structural, cellular and molecular biology. Ann Rev Biochem. 2000; 69: 145-52.
Umamaheswari M, Madeswaran A, Asokkumar K, Sivashanmugam T, Subhadradevi V, Jagannath P. Discovery of potential xanthine oxidase inhibitors using in silico docking studies. Der Pharma Chemica. 2011; 3: 240-47.
Vane JR, Bakhle YS, Botting RM. Cyclooxygenases 1 and 2. Annu Rev Pharmacol Toxicol. 1998; 38: 97-120.
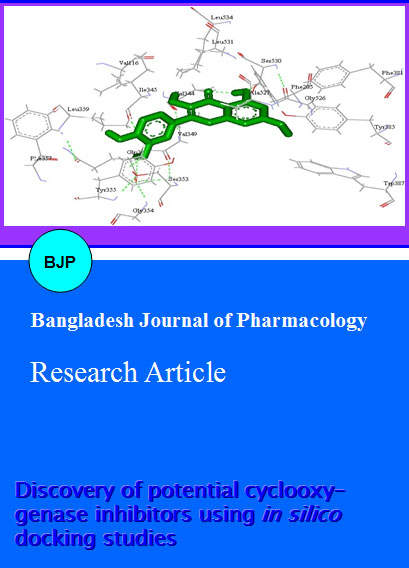