Dipeptidyl peptidase IV inhibitors derived from a mangrove flora Rhizophora mucronata: An in silico approach
Abstract
Dipeptidyl peptidase IV (DPP IV) is responsible for conversion of glucose tolerance (GLP-1), into inactive form. The inhibition of DPP-IV would be beneficial in the treatment of diabetes mellitus. Therefore, the aim of the present study was to isolate and evaluate cystine, phenyl acetic acid, acrylamide, caprylone and oleic acid from Rhizophora mucronata inhibitory action on DPP IV inhibitors using in silico approach. In silico analysis of cystine, phenyl acetic acid, acrylamide, caprylone and oleic acid on human apo DPP IV protein was done by using AutoDock 4.0. Among the five compounds cysteine acts as an inhibitor with binding energy -5.9 kcal/mol, seven hydrogen bond interactions at residues VAL459, VAL 459, GLU408, GLU206, ARG358, GLU205 and SER209 to suppresses the action of DPP IV protein.
Introduction
Diphenyl peptidase IV (DPP IV) is a serine peptidases plays vital role in regulation of incretins hormone (Figure 1). This pleiotropic enzyme inactivates two intestinal hormones glucagon like peptide-1 (GLP-1) and glucose-dependent insulinotropic polypeptide (GIP) both are well known as incretins (Abbott, 2000). The GLP-1 stimulates insulin secretion and beta cell function leads to decreases glucagon secretion by lowering the blood glucose level (Weber, 2004). However, incretins have very short half-lives due to renal degradation and proteolytic cleavage by dipeptidyl peptidase. To extend the incretin effect on enhanced insulin secretion, two approaches can be taken, the intravenous administration of GLP-1 or the oral administration of DPP IV inhibitors to slow down the otherwise rapid inactivation of endogenous GIP and GLP-1 (Mest and Mentlein, 2005). Compare to GLP-1 analogues, DPP IV inhibitors are orally bioavailable and cause temporary side effects. Over the past few decades, synthetic peptide-derived DPP IV inhibitors including Vildagliptin, Saxagliptin and Sitagliptin had been approved for management of type 2 diabetes mellitus in Western countries. DPP IV inhibitors seem to represent an resourceful new class of oral normoglycemic agents, with a potential effect on pancreatic function, but the real efficacy and safety have to be firmly assessed in the future (Alexandre Fredenrich et al., 2009). Rather the DPP IV inhibitors would fit into the typical algorithms for treatment of type 2 diabetes mellitus remains a matter for debate. For safety and cost effective measures, Diabetologist focussed their interest towards DPP IV inhibitor from potentially available medicinal plants through in silico approaches. The effects of bioactive drugs from floral system delay the development of diabetic complications and correct the metabolic abnormalities with more efficacy than hypoglycemic agents used in clinical therapy (Bnouham et al., 2007).
Rhizophora mucronata is a mangrove grows in the tropical and subtropical region coastlines of Southeast Asia (Rohini and Das, 2009). Historically mangroves, has been used as traditional medicine in the treatment of diarrhea, dysentery, blood in urine, fever and gastric disorder (Mangrove and wetland wildlife, 2007; Bandaranayake, 1999). Formulation and validation of floral chemicals used as a drug is a pinpoint in the field of medicinal chemistry (Borges et al., 2002). The foremost objective of drug design is to improve efficacy, potency and to minimize for eliminate untoward side effects. Bioinformatics tools play a vital role to identifying specific drug targets. The target structures for possible binding sites, generation of candidate molecules, checking for their drug likeness, docking the molecules with the target, rank them according to their binding affinities, and further optimization of the molecules to improve binding characteristics analysed using bioinformatics tool (Breda et al., 2008). AutoDock 4.2 is a complement of automated docking tool starts with the definition of a binding site, in general a restricted region of the protein. AutoDock uses Monte Carlo and Simulated Annealing in combination with Genetic Algorithm which is used for global optimization (Morris et al., 1998). The antidiabetic activity of Citrullus colocynthis (Gurudeeban et al., 2010), R. mucronata (Ramanathan et al., 2008), Aegiceras corniculatum (Gurudeeban et al., 2012) and alpha glucosidase effect of coastal medicinal plants (Gurudeeban et al., 2012) were scientific evidence on glucose control of coastal medicinal plants. But, there is paucity of scientific data of R. mucronata on DPP IV inhibitory effect. Hence the present study, we investigated in silico docking of phytocompounds cystine, phenylacetic acid, acrylamide, caprylone and oleic acid from R. mucronata as DPP IV inhibitor.
Figure 1: Cleavage site of GLP-1 protein by DPP4 (The yellow colour marking indicates DPP4 interrupting point of GLP-1)
Materials and Methods
Preparation of coordinate file
An extended PDB format, termed as PDBQT file was used for coordinate files which includes atomic partial charges. Auto Dock Tools was used for creating PDBQT files from traditional PDB files. Three dimensional structure of human apo DPP IV (PDB: 1PFQ) was obtained from Protein Data Bank (Figure 2). This structure was determined using X-ray Diffraction. Cystine, phenyl acetic acid, acrylamide, caprylone and oleic acid were isolated from R. mucronata, identified using Gas chromatographymass spectroscopy and deposited in PubChem (Gurudeeban et al., 2012). The 2D structure of isolated compounds was retrieved from NCBI PubChem Compound database in SDF format and was then converted to PDB format using OPEN BABEL 2.2.119 (Table I). The 2D structure of the ligands was shown in Figure 3. The optimized ligand molecules were docked into distinguished Cystine, phenyl acetic acid, acrylamide, caprylone and oleic acid model using Ligand Fit in the Auto Dock 4.0 (Khodade et al., 2007).
Table I: Ligand used for AutoDock
Ligand | Smiles | PubChem ID | Hydrogen donor and acceptor | Molecular weight |
---|---|---|---|---|
Cystine | C(C(C(=O)O)N)SSCC(C(=O)O)N | CID 595 | 4,8 | 240.3 |
Phenyl acetic acid | C1=CC=C(C=C1)CC(=O)O | CID 999 | 1,2 | 136.1 |
Acrylamide | C=CC(=O)N | CID 6579 | 1,1 | 71.1 |
Caprylone | CCCCCCCC(=O)CCCCCCC | CID 13162 | 0,1 | 226.4 |
Oleic Acid | CCCCCCCCC=CCCCCCCCC(=O)O | CID 445639 | 1,2 | 282.5 |
Figure 2: 3D structure of human apo dipeptidyl peptidase-4 protein
Figure 3: 2D structure of phytocompounds of R. mucronata (A) cystine (B) phenyl acetic acid (C) acrylamide (D) caprylone (E) oleic acid. The structure retrieved from PubChem database
In silico analysis
AutoDock Tools 4.0 was used to prepare, run, and analyze the docking simulations. Polar hydrogens, Kollman charges, and solvation parameters were added into the (Protein) receptor PDB file for the preparation of protein in docking simulation. The precalculated grid maps, one for each atom type present in the flexible molecules being docked and its stores the potential energy arising from the interaction with rigid macromolecules. This grid must surround the region of interest in the rigid macromolecule. The grid box size was set at 60, 60 and 60 A° (x, y, and z) to include all the amino acid residues that present in rigid macromolecules which were used to produce grid maps by Auto Dock 4.0. The spacing between grid points was 0.451 angstroms. The Lamarckian Genetic Algorithm (LGA) 23 was chosen search for the best conformers. Maximum of 10 conformers was considered to the docking process. The population size was set to 150 and the individuals were initialized randomly. Maximum number of energy evaluation was set to 250000, maximum number of generations 54000, maximum number of top individual that automatically survived set to 1, mutation rate of 0.02, crossover rate of 0.8, Step sizes were 0.2Aº for translations, 5.0° for quaternions and 5.0° for torsions. Cluster tolerance 0.5Aº, external grid energy 1000, max initial energy 0.0, max number of retries 10000 and 10 LGA runs were performed. Auto Dock was compiled and run under Windows XP operating system. AutoDock results were analyzed to study the interactions and the binding energy of the docked structure. All the Auto Dock docking runs were performed in Intel CORETM i5, 64 bit Operating System and 4GB RAM in Lenovo Win 7 PC.
Determination of binding energy
Binding energy is an important problem for structure based drug design. The correlation of tentative and predicted protein-ligand binding energies were calculated using the following formula,
Binding energy = FIE + VDE + HB + DE + EsE+ FTIE + TFE + USE (kcal/mol)
Whereas,
[FIE- final intermolecular energy; VDE- Van Der Waals energy; HB- hydrogen bonds; DE- desolvation energy; EsE + electrostatic energy; FTIE-Final total internal energy; TFE- Torsional free energy; USE-Unbound system energy] (Zhang et al., 2008).
Distinct conformational clusters RMSD-tolerance and Van der Waals scaling factor was found to be 2.0…. 1.0 Aº… respectively.
Results
Mangrove derived phytocompounds were evaluated to control type 2 diabetes to enhance the activity of GLP-1 and GIP by inhibiting DPP IV enzyme activity in the first time of computational scientific validation. R. mucronata derived ligands such as cystine, phenyl acetic acid, acrylamide, caprylone and oleic acid were subjected to docking analysis with human apo DPP IV using AutoDock 4.0. The docking poses were ranked according to their docking scores and both the ranked list of docked ligands and their corresponding binding poses (Morris et al., 1998). 10 docking runs were performed. Grid parameters were set as mentioned earlier and spacing between grid points was 0.375Aº…. After the simulations were complete, the docked structures were analyzed and the interactions were observed. Hydrogen bond interactions and the binding distance between the donors and acceptors were measured for the best conformers. Interaction free energies are crucial to analyze binding propensities in proteins. While the problem of computing binding free energies remains open, approximate estimates have become very useful for filtering potential binding complexes. Based on the low binding energies the ligand molecules were selected and identified as potential therapeutic action having sources.
Molecular interaction of DPP IV with R. mucronata derived phytocompounds
Interaction with cystine
Docking simulation of R. mucronata derived cystine into DPP IV A chain produced six clusters of conformers using Root mean square difference (RMSD) -tolerance of 2.0Aº… out of 10 docking runs. Cluster Rank 1 with binding energy -5.8 kcal/mol at 8th run has three hydrogen bond interactions at residues VAL459, VAL 459 and GLU408 with cluster RMSD 0.0 and reference RMSD22.6 (Table II and Figure 4). Similarly cystine into DPP IV B chain produced six clusters with binding energy -5.9 kcal/mol at 3rd run has four hydrogen bond interactions at residues GLU206, ARG358, GLU205 and SER209 with reference RMSD22. 46.8 (Table III and Figure 4). Hydrogen bond distance between the donor and acceptor atoms was found to be 2.0, 2.0, 1.7 in chain A and 2.1, 2.0, 1.3 and 2.0 in chain B respectively.
Table II: Molecular interactions of phytocompounds on DPP IV (A Chain)
Docked Molecule | No. of Hydrogen bonds | Hydrogen bond donor | Hydrogen bond acceptor | Length of Hydrogen bond (A) | Binding energy | Cluster RMSD | Reference RMSD |
---|---|---|---|---|---|---|---|
Cystine | 3 | VAL459:HN VAL 459: O GLU408:OE1 |
LIG: A: CYS1: O LIG:A:CYS1:H2 LIG: A: CYS1:H1 |
2.0 2.0 1.7 |
-5.8 | 0.0 | 22.6 |
Phenyl acetic acid | 3 | ARG356:HN PHE357:HH ARG358:HN |
LIG1: O LIG1: O LIG1: O |
2.0 2.0 2.1 |
-3.8 | 0.0 | 18.2 |
Acrylamide | 3 | GLU206: O ARG669:HH27 VAL207: O |
LIG1:H LIG1: O LIG1:H |
2.0 1.9 2.1 |
-3.2 | 0.0 | 11.6 |
Caprylone | 1 | VAL303:HN | LIG1: O | 2.0 | -3.2 | 1.9 | 17.8 |
Oleic Acid | 2 | ARG125:HH11 ARG125:HH21 |
LIG1: O LIG1: O |
1.9 2.1 |
-2.5 | 0.0 | 11.5 |
Table III: Molecular interactions of phytocompounds on DPP IV (B Chain)
Docked Molecule | No. of Hydrogen bonds | Hydrogen bond donor | Hydrogen bond acceptor | Length of hydro-gen bond (A) | Binding energy | Cluster RMSD | Reference RMSD |
---|---|---|---|---|---|---|---|
Cystine | 4 | GLU206:OE1 ARG358:HH22 GLU205: O SER209: HG |
CYS1:H1 CYS1:OXT CYS1:H2 CYS1: O |
2.1 2.0 1.3 2.0 |
-5.9 | 0.0 | 46.8 |
Phenyl acetic acid | 2 | ARG356:HN PHE357:HN |
LIG1: O LIG1: O |
1.7 2.0 |
-4.0 | 0.1 | 57.4 |
Acrylamide | 2 | GLU206: O PHE357: O |
LIG1:H LIG1:H |
2.2 2.0 |
-3.1 | 6.0 | 51.4 |
Caprylone | 2 | TRY211:HN SER212:HN |
LIG1: O LIG1: O |
2.1 2.0 |
-3.8 | 0.0 | 45.0 |
Oleic Acid | 2 | LYS554:H21 ASN562:HD22 |
LIG1: O LIG1: O |
1.9 2.0 |
-3.1 | 0.0 | 41.0 |
Figure 4: Inhibitory action of cystine with DPP-IV using AutoDock hydrogen bond interaction A) A chain B) B chain
Interaction with phenyl acetic acid
Docking simulation of phenyl acetic acid into DPP IV A chain produced single cluster of conformers using RMSD-tolerance of 2.0 Aº out of 8th docking runs. Cluster Rank 1 with binding energy -3.8 kcal/mol at 8th run has formed three hydrogen bond interactions at residue ARG356, PHE357 and ARG358 with reference RMSD 18.2 (Table II and Figure 5). Accordingly, Phenyl acetic acid into DPP IV B chain produced single cluster with binding energy -4.0 kcal/mol at 7th run has formed two hydrogen bond interaction at residue ARG356, PHE357 and reference RMSD 57.4 (Table III and Figure 5). Hydrogen bond distance between the donor and acceptor in chain A, B was found to be 2.0, 2.0, 2.1 and 1.7, 2.0 respectively.
Interaction with acrylamide
Docking simulation of acrylamide into DPP IV A chain produced single cluster of conformers using RMSD-tolerance of 2.0Aº out of 8th docking runs. Cluster Rank 1 with binding energy -3.2 kcal/mol at 8th run has formed three hydrogen bond interaction at residue GLU206, ARG669, VAL207 with cluster RMSD 0.0 and reference RMSD 11.6 (Table II and Figure 6). Also, B chain produced single cluster with binding energy -3.1 kcal/mol at 9th run has formed two hydrogen bond interaction at residue GLU206, PHE357 and reference RMSD 51.4 (Table III and Figure 6). Hydrogen bond distance between the donor and acceptor of chain A, B was found to be Hydrogen bond distance between the donor and acceptor was found to be 2.0, 1.9 and 2.1, 2.2, and 2.0.
Figure 5: Inhibitory action of phenyl acetic acid with DPP-IV using AutoDock hydrogen bond interaction A) A chain B) B chain
Figure 6: Inhibitory action of acrylamide with DPP-IV using AutoDock hydrogen bond interaction A) A chain B) B chain
Interaction with caprylone
Docking simulation of caprylone into DPP IV B chain produced single cluster of conformers using RMSD-tolerance of 2.0Aº out of 5th docking runs. Cluster Rank 1 with binding energy -3.2 kcal/mol at 1st run has formed one hydrogen bond interaction at residue VAL303 with cluster RMSD 1.9 and reference RMSD 18.0 (Table II and Figure 7). Caprylone into DPP IV B chain produced single cluster with binding energy -3.8 kcal/mol at 9th run has formed two hydrogen bond interactions at residue TRY211, SER212 with reference RMSD 45.0 (Table II and Figure 7). Hydrogen bond distance between the donor and acceptor was found to be 2.0, 2.1 and 2.0.
Interaction with oleic acid
Docking simulation of oleic acid into DPP IV, a chain produced single cluster of conformers using RMSD-tolerance of 2.0Aº out of 5th docking runs. Cluster Rank 1 with binding energy -2.4 kcal/mol at 5th run has formed two hydrogen bond interaction at residue ARG125 with cluster RMSD 0.0 and reference RMSD 11.4 (Table II and Figure 8). Also, into DPP IV B chain interact with oleic acid produced single cluster with binding energy-3.1 kcal/mol at 6th run has formed two hydrogen bond interaction at residue LYS554, ASN562 and reference RMSD 41.0 (Table III and Figure 8). Hydrogen bond distance between the donor and acceptor was found to be 1.9 and 2.1, 1.9 and 2.0.
Figure 7: Inhibitory action of caprylone with DPP-IV using AutoDoc hydrogen bond interaction A) A chain B) B chain
Figure 8: Inhibitory action of oleic acid with DPP-IV using AutoDoc hydrogen bond interaction A) A chain B) B chain
Discussion
DPP IV is a member of serine peptidase plays a role of inhibitory action to increase blood concentration of the incretin GLP-1. Development of small molecule as selective inhibitors of DPP IV is a major challenge. Natural DPP IV inhibitors like berberine, group of isoquinoline alkaloids, reported an effective inhibition against the DPP IV enzyme (Chakrabarti et al., 2011). Current DPP IV drugs in market vildagliptin, sitagliptin and saxagliptin had numerous side effects like tremor, headache, dizziness, low blood sugar levels specially when taken in excess of amount, nausea, feeling weak, weight gain and swelling of the legs and ankles due to excess fluid retention (John and White, 2008). These drugs have to be taken as combination therapy with natural therapeutic molecule to achieve desired results. Earlier, the extracts from Armenian Highland plants was highly effective in inhibiting DPP IV was reported by St. John's wort and sea-buckthorn leaves could be used, in combination with other antidiabetic drugs, for the treatment of diabetes mellitus. The present study results have been integrated with numerous natural products indoline form of arylaminoprolylthiazolidine, quinolone derivative of tetrahydro-2H-11-oxa-2, 4, 10-triaza-benzo[b]fluoren-1-one compounds are potent antidiabetic agents through a DPP IV inhibition mechanism (Sakashita et al., 2007; Balajee and Dhanarajan, 2012; Kumar et al., 2012). Cystine is semi essential amino acids found in most high-protein foods. Plants like red peppers, garlic, onions, broccoli, Brussels sprout, oats, granola, wheat germ and sprouted lentils contains more amount of cystine (Weast and Robert, 1981). Previous studies indicated cystine has antiviral, antimicrobial and anticancer properties (Kiel et al., 2000). Our present result is a new approach to assess antidiabetic molecule in the form of DPP IV inhibitors.
In conclusion, cystine interacts into DPP IV at VAL459, VAL 459, GLU408, GLU206, ARG358, GLU205 and SER209 forming three hydrogen bonds in A chain and four hydrogen bonds in B chain with high affinity. These sites could be the best possible binding sites to inhibit the human apo DPP IV protein.
Conclusion
Acknowledgements
The authors are gratefully acknowledged to the Director & Dean, Faculty of Marine Sciences, Annamalai University, Parangipettai, Tamil Nadu and University Grant Commission, New Delhi, India for providing all support during the study period.
References
Abbott CA, Yu DMT, Woollatt E, Sutherland GR, McCaughan GW, Gorrell MD. Cloning, expression and chromosomal localization of a novel human dipeptidyl peptidase (DPP) IV homolog, DPP8. Eur J Biochem. 2000; 67: 6140-50.
Balajee R, Dhanarajan MS. Identification and comparative molecular docking analysis of 6, 7, 8, 9-tetrahydro-2H-11-oxa-2,4,10-triazabenzo[B] fluoren-1-one (RBMS-01) bounds with DPP4 for antihyperglycemic activities. Chem Sci Transc. 2012; 1: 279-88.
Bandaranayake WM. Economic, traditional and medicinal uses of mangroves. Australian Institute of Marine Science, Town-sville, Australia, 1999, p 82.
Bnouham M, Ziyyat A, Mekhfi H, Tahri A, Legssyer A. Medicinal plants with potential antidiabetic activity: A review of ten years of herbal medicine research. Int J Diabetes Metab. 2006; 14: 1-25.
Borges BF, Jorge MR, Morgan CM, Da Silveria DX, Custodio O. Binge-eating disorder in Brazilian women on a weight-loss program. Obesity Res. 2002; 10: 1127-34.
Breda A, Basso LA, Santos DS, De Azevedo JR. Virtual screening of drugs: Score functions, docking and drug design. Curr Comput Aided Drug Des. 2008; 4: 265-72.
Fredenrich A, Palle S, Canivet B. Dipeptidyl peptidase inhibitors: A new step towards normoglycemia. Open Endocrinol J. 2009; 3: 16-21.
Gurudeeban S, Ramanathan T. Antidiabetic effect of Citrullus colocynthis in alloxan-induced diabetic rats. Inventi Rapid Ethnopharmacol. 2010; 1: 112.
Gurudeeban S, Satyavani K, Ramanathan T, Balasubramanian T. Antidiabetic effect of a black mangrove species Aegiceras corniculatum in alloxan-induced diabetic rats. J Adv Pharm Technol Res. 2012; 3: 52-56.
Gurudeeban S, Satyavani K, Ramanathan T. Alpha glucosidase inhibitory effect and enzyme kinetics of coastal medicinal plants. Bangladesh J Pharmacol. 2012; 7: 186-91.
Khodade P, Prabhu R, Chandra N, Raha S, Govindarajan R. Parallel implementation of AutoDock. J Applied Crystallogr. 2007; 40: 598-99.
Kiel S, Bruss M, Bonisch H, Gothert M. Pharmacological properties of the naturally occurring phe-124-cys variant of the human 5-HT1B receptor: Changes in ligand binding, G-protein coupling and second messenger formation. Pharmacogenetics 2000; 10: 655-66.
Kumar S, Kumar V, Rana M, Kumar D. Enzymes inhibitors from plants: An alternate approach to treat diabetes. Pharmaco Commun. 2012; 2: 18-33.
Mest HJ, Mentlein R. Dipeptidyl peptidase inhibitors as new drugs for the treatment of type 2 diabetes. Diabetologia 2005; 48: 616-20.
Morris GM, Goodsell DS, Halliday RS, Huey R, Hart WE, Belew RK, Olson AJ. Automated docking using a Lamarckian genetic algorithm and an empirical binding free energy function. J Comput Chem. 1998; 19: 1639-62.
Ramanathan T, Hariharan B, Ganesan K. Antidiabetic activity of a coastal mangrove leaves of Rhizophora mucronata. Int. J Plant Res Plant Arch. 2008; 8: 931-33.
Rohini RM, Das AK. A comparative evaluation of an analgesic and anti-inflammatory activities of I Rhizophora mucronata bark extracts. Pharmacol Online. 2009; 1: 780-91.
Sakashita H, Akahoshi F, Yoshida T, Kitajima H, Hayashi Y, Ishii S, Takashina Y, Tsutsumiuchi R, Ono S. Lead optimization of [(S)-gamma-(arylamino)prolyl]thiazolidine focused on gamma-substituent: Indoline compounds as potent DPP-IV inhibitors. Bioorg Med Chem. 2007; 15: 641–55.
Weast RC (ed). CRC handbook of chemistry and physics. 62nd ed. Boca Raton, FL, CRC Press, 1981, p C-259.
Weber AE. Dipeptidyl peptidase IV inhibitors for the treatment of diabetes. J Med Chem. 2004; 47: 4135-41.
Zhang S, Kumar K, Jiang X, Wallqvist A, Reifman J. DOVIS: An implementation for high-throughput virtual screening using AutoDock. BMC Bioinform. 2008; 9: 126.
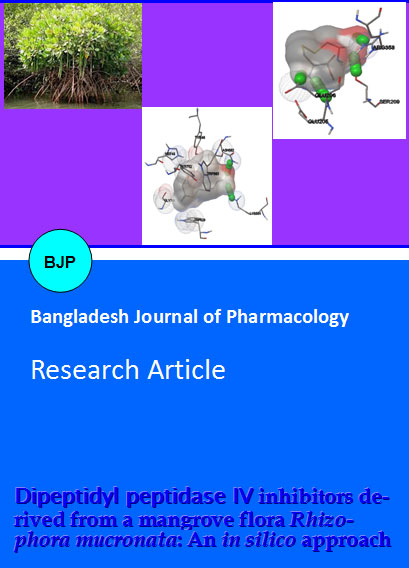