In silico docking studies of aldose reductase inhibitory activity of commercially available flavonoids
Abstract
The primary objective of this study was to investigate the aldose reductase inhibitory activity of flavonoids using in silico docking studies. In this perspective, flavonoids like biochanin, butein, esculatin, fisetin and herbacetin were selected. Epalrestat, a known aldose reductase inhibitor was used as the standard. In silico docking studies were carried out using AutoDock 4.2, based on the Lamarckian genetic algorithm principle. The results showed that all the selected flavonoids showed binding energy ranging between -9.3 to -7.2 kcal/mol when compared with that of the standard (-8.7 kcal/mol). Inhibition constant (144.1 to 5.0 µM) and intermolecular energy (-11.4 to -7.8 kcal/mol) of the flavonoids also coincide with the binding energy. All the selected flavonoids contributed aldose reductase inhibitory activity because of its structural properties. These molecular docking analyses could lead to the further development of potent aldose reductase inhibitors for the treatment of diabetes.
Introduction
Diabetes mellitus is a metabolic disorder characterized by hyperglycemia resulting from defects in insulin action, insulin secretion or both. Type 1 diabetes is caused by a deficiency of beta-pancreatic cells insulin secretion. Type 2 diabetes is associated with obesity and is characterized by an initial phase progressive insulin resistance, with ensuing reduction in the ability of pancreatic hormone to promote peripheral glucose disposal and to decreases hepatic glucose output (Vianna et al., 2011; Lamba et al., 2011).
Flavonoids and their related compounds are low molecular weight substances, which are a group of natural products which exhibits various biological and pharmacological activities like antibacterial, antiviral, antioxidant, anti-inflammatory, anti-allergic, hepatoprotective, antithrombotic, antimutagenic effects and inhibition of several enzymes have also been investigated (Cushnie and Lamb, 2011; Gonzalez and Ballester, 2011; Nishiumi and Miyamoto, 2011; Liu et al., 2007).
Aldose reductase (ALR2; EC: 1.1.1.21) belongs to aldo-keto reductases super family. It is the first rate limiting enzyme in polyol pathway and reduces glucose to sorbitol by utilizing NADPH as a cofactor. Sorbitol dehydrogenase is the enzyme responsible for the conversion of sorbitol into fructose (Ravindranath et al., 2009; Hwang et al., 2005). The polyol pathway represents a minor route of glucose utilization, accounting for less than 3% of glucose consumption. However, in the presence of high glucose, the activity of this pathway is increased and could represent up to 30% of total glucose consumption (Yadav et al., 2009). Abnormal activation of the polyol pathway during diabetes leads to accumulation of osmotically active sorbitol leading to osmotic as well as oxidative stress, resulting in tissue injury (Saraswat et al., 2008; Dong et al., 2005). Evidence for the involvement of ALR2 in diabetic neuropathy, retinopathy, nephropathy and cataract emerged from several independent studies (Guzman and Guerrero, 2005).
Molecular docking is a frequently used tool in computer-aided structure-based rational drug design. It evaluates how small molecules called ligands (flavonoids) and the target macromolecule (aldose reductase enzyme) fit together (Norgan et al., 2011; Seeliger and de GrootLigand, 2010). AutoDock Tools (ADT) is a program package of automated docking tools and designed to predict how small molecules bind to a target protein of known 3D-structure. Besides generating binding energies in these docking studies, the position of the ligand in the enzyme binding site can be visualized (Zhang et al., 2008). It can be useful for developing potential drug candidates and also for the understanding the binding nature. The objective of the present work is to study the in silico aldose reductase inhibitory activity of commercially available flavonoids.
Materials and Methods
Softwares required
Python 2.7- language was downloaded from www.python.com, Cygwin (a data storage) c:\program and Python 2.5 were simultaneously downloaded from www.cygwin.com, Molecular graphics laboratory (MGL) tools and AutoDock4.2 was downloaded from www.scripps.edu, Discovery studio visualizer 2.5.5 was downloaded from www.accelerys.com, Molecular orbital package (MOPAC), ChemSketch was downloaded from www.acdlabs.com. Online smiles translation was carried out using cactus.nci.nih.gov/translate/.
Docking methodology
Lamarckian genetic algorithm (LGA) for ligand conformational searching is used for the docking, which is a hybrid of genetic algorithm and local search algorithm. This algorithm first builds a population of individuals, each being a different random conformation of the docked molecule. Each individual is then mutated to acquire a slightly different translation and rotation and the local search algorithm then performs energy minimizations on a user-specified proportion of the individuals. The individuals with the low resulting energy are transferred to the next generation and the process is then repeated. The algorithm is called Lamarckian genetic algorithm because every new generation of individuals is allowed to inherit the local search adaptations of their parents (Madeswaran et al., 2011).
An extended PDB format, termed as PDBQT file was used for coordinate files which includes atomic partial charges. AutoDock Tools was used for generating PDBQT files from traditional PDB files (Umamaheswari et al., 2012). Crystal structure of aldose reductase enzyme was downloaded from the Brookhaeven protein data bank (Figure 1).
Even though there are thousands of structures of aldose reductase enzymes are present in the database but the 3EL3 were selected for the present study due to its similarity with the human enzyme. Albaflavenone synthase (CYP170A1) is monooxygenase catalyzing the final two steps in the biosynthesis of this antibiotic in the soil bacterium, Streptomyces coelicolor A3. Interestingly, CYP170A1 shows no stereo selection forming equal amounts of two albaflavenol epimers, each of which is oxidized in turn to albaflavenone. To explore the structural basis of the reaction mechanism, we have studied the crystal structures of both ligand-free CYP170A1 (2.6 A) and complex ofendogenous substrate (epi-isozizaene) with CYP170A1 (3.3 A). The structure of the complex suggests that the proximal epi-isozizaene molecules may bind to the heme iron in two orientations.
In Figure 2, the flavonoid ligands like biochanin, butein, esculatin, fisetin, herbacetin and epalrestat were built using ChemSketch and optimized using "Prepare Ligands" in the AutoDock 4.2 for docking studies. The optimized ligand molecules were docked into reï¬ned aldose reductase model using “LigandFit†in the AutoDock 4.2 (Umamaheswari et al., 2011).
Lead optimization of the selected compounds was done by computation of druglikeness properties. The druglikeness scores of the compounds were evaluated with the help of Lipinski's rule. The various parameters of the ligands like molecular formula, molecular weight, aromatic carbons, rotatable bonds and number of torsions were tabulated in Table I.
Table I: Ligand parameters
Ligands | Molecular formula | Molecular weight | Aromatic carbons | Rotatable bonds | Number of torsions |
---|---|---|---|---|---|
Biochanin | C16H12O5 | 284.3 | 15 | 4 | 4 |
Butein | C15H12O5 | 272.3 | 12 | 7 | 7 |
Esculatin | C9H6O5 | 178.1 | 9 | 2 | 2 |
Fisetin | C15H10O6 | 286.2 | 15 | 5 | 5 |
Herbacetin | C15H10O7 | 302.2 | 15 | 6 | 6 |
Epalrestat | C15H13NO3S2 | 319.4 | 9 | 5 | 5 |
The enzyme was refined using various steps with the help of Accelrys studio viewer. The water molecules and heavy metal atoms have been removed from the enzyme and then hydrogen and Kollman charges has been added to the enzyme. Then the pdb format of the enzyme converted into suitable pdbqt format for the docking.
The preparation of the target protein 1EL3 with the AutoDock Tools software involved adding all hydrogen atoms to the macromolecule, which is a step necessary for correct calculation of partial atomic charges. Gasteiger charges are calculated for each atom of the macromolecule in AutoDock 4.2 instead of Kollman charges which were used in the previous versions of the program. Three-dimensional affinity grids of size 277 x 277 x 277 Angstrom with 0.6 Angstrom spacing were centered on the geometric center of the target protein and were calculated for each of the following atom types: HD, C, A, N, OA, and SA, representing all possible atom types in a protein. Additionally, an electrostatic map and a desolvation map were also calculated (Umamaheswari et al., 2011). The dimensional affinity grid was fixed based upon the necessity to run the AutoGrid file.
Rapid energy evaluation was achieved by precalculating atomic affinity potentials for each atom in the ligand molecule. In the AutoGrid procedure, the target enzyme was embedded on a three dimensional grid point (Madeswaran et al., 2011). The energy of interaction of each atom in the ligand was encountered.
The selected important docking parameters for the LGA as follows: Population size of 150 individuals, 2.5 million energy evaluations, maximum of 27000 generations, number of top individuals to automatically survive to next generation of 1, mutation rate of 0.02, crossover rate of 0.8, 10 docking runs, and random initial positions and conformations. The probability of performing local search on an individual in the population was set to 0.06 (Umamaheswari et al., 2011).
AutoDock was run several times to get various rigid docked conformations, and used to analyze the predicted docking energy. The binding sites for these molecules were selected based on the ligand-binding pocket of the templates. AutoDock Tools provide various methods to analyze the results of docking simulations such as, conformational similarity, visualizing the binding site and its energy and other parameters like intermolecular energy and inhibition constant. For each ligand, ten best poses were generated and scored using AutoDock 4.2 scoring functions (Madeswaran et al., 2012).
Result and Discussion
Analysis of the receptor/ligand complex models generated after successful docking of the flavonoids was based on the parameters such as hydrogen bond interactions, pai-pai interactions, binding energy, RMSD of active site residues and orientation of the docked compound within the active site (Azam et al., 2011, Zhang et al., 2008).
The docking poses were ranked according to their docking scores and both the ranked list of docked ligands based on their binding energy with the enzyme and their corresponding binding poses (Madeswaran et al., 2011). In Figure 3, docked pose of aldose reductase enzyme with the ligands butein and epalrestat clearly demonstrated the binding positions of the ligand with the enzyme.
In silico docking study, was carried out to identify the inhibiting potential of selected flavonoids against aldose reductase enzyme. In this study different flavonoids were selected for the in silico docking studies. Lead optimization of the selected compounds was done by computation of druglikeness properties. The druglikeness scores of the compounds were evaluated with the help of Lipinski's rule. The docking studies were performed by the use of AutoDock 4.2. In the docking studies, if a compound shows lesser binding energy compared to the standard it proves that higher possibility of the compound has higher activity.
The binding sites of the epalrestat was found to be GLY 18, TRP 20, LYS 21, TYR 48, TRP 111, TYR 209, SER 210, SER 214, ILE 260. The potential binding sites of the butein was found that, GLY 18, TRP 20, GLN 26, VAL 27, TRP 111, PHE 122, TYR 209, SER 210, ILE 260. This proves that the effective binding sites are present in the selected flavonoid butein when compared with the standard epalrestat. It proves that the ability of inhibiting the aldose reductase enzyme by the selected flavonoids.
Binding energy of the individual compounds were calculated by using the following formula,
Binding energy = A + B + C - D
Where, A denotes final intermolecular energy + van der Walls energy (vdW) + hydrogen bonds + desolvation energy + electrostatic energy (kcal/mol), B denotes final total internal energy (kcal/mol), C denotes torsional free energy (kcal/mol), D denotes unbound system's energy (kcal/mol).
As shown in Table II, flavonoids showed binding energy ranging between -9.3 to -7.2 kcal/mol. All the selected flavonoids had showed better and consistent binding energy when compared to standard epalrestat (-8.7 kcal/mol). This proves that flavonoids consist of potential aldose reductase inhibitory binding sites similar to that of the standard.
Table II:Binding energies of the compounds based on their rank
Compound | Binding energies of the compounds based on their rank (kcal/mol) | |||||||||
---|---|---|---|---|---|---|---|---|---|---|
1 | 2 | 3 | 4 | 5 | 6 | 7 | 8 | 9 | 10 | |
Biochanin | -8.9 | -8.9 | -8.9 | -8.9 | -8.9 | -8.9 | -8.9 | -8.7 | -7.8 | -7.8 |
Butein | -9.3 | -8.9 | -8.8 | -8.5 | -8.4 | -7.9 | -9.0 | -8.1 | -7.4 | -7.0 |
Esculatin | -7.2 | -7.2 | -7.2 | -7.2 | -7.2 | -7.2 | -7.2 | -7.2 | -7.2 | -7.1 |
Fisetin | -8.7 | -8.7 | -8.6 | -8.5 | -8.2 | -8.1 | -8.7 | -8.1 | -8.0 | -8.4 |
Herbacetin | -8.8 | -7.6 | -6.6 | -6.6 | -6.5 | -6.5 | -6.5 | -6.4 | -6.4 | -6.1 |
Epalrestat | -8.7 | -8.6 | -8.6 | -8.1 | -7.9 | -7.8 | -7.6 | -7.2 | -7.7 | -7.1 |
In addition, two other parameters like inhibition constant (Ki) and intermolecular energy were also determined. Inhibition constant is directly proportional to binding energy. As shown in Table III, flavonoids showed inhibition constant ranging from 144.1 to 5.0 µM. All the selected compounds had lesser inhibition constant when compared to the standard (397.2 nM). Thus, the aldose reductase inhibitory activity of the flavonoids were compared with the standard.
Table III: Inhibition Constant of the compounds based on their rank
Compound | Inhibition Constant of the compounds based on their rank (mM, aµM) | |||||||||
---|---|---|---|---|---|---|---|---|---|---|
1 | 2 | 3 | 4 | 5 | 6 | 7 | 8 | 9 | 10 | |
Biochanin | 300.2 | 302.1 | 306.5 | 307.8 | 308.7 | 313.4 | 321.5 | 412.5 | 1.9a | 2.0a |
Butein | 144.1 | 309.6 | 349.3 | 565.7 | 652.7 | 1.5a | 266.2 | 1.1a | 4.0a | 7.6a |
Esculatin | 5.0a | 5.0a | 5.0a | 5.2a | 5.3a | 5.4a | 5.4a | 5.6a | 5.6a | 5.7a |
Fisetin | 405.0 | 425.7 | 461.1 | 554.2 | 1.0a | 1.2a | 408.7 | 1.1a | 1.3a | 662.8 |
Herbacetin | 332.0 | 2.5a | 14.1a | 14.6a | 15.7a | 15.7a | 15.8a | 19.5a | 21.1a | 31.2a |
Epalrestat | 397.2 | 458.0 | 469.2 | 1.1a | 1.7a | 1.8a | 2.7a | 5.0a | 2.3a | 6.6a |
Intermolecular energy is also directly proportional to binding energy. As shown in Table IV, flavonoids showed intermolecular energy ranging between -11.4 to -7.8 kcal/mol which was lesser when compared to the standard (-10.5 kcal/mol). We found a decrease in intermolecular energy of all the selected compounds with a simultaneous decrease in the binding energy. This result further proved the aldose reductase inhibitory activity of all the selected flavonoids. Based on the current docking studies, the aldose reductase inhibitory activity of the selected compounds was found to be decreased in the order of butein, biochanin, herbacetin, epalrestat, fisetin, esculatin. On the basis of the above study, all the selected flavonoids showed better aldose reductase inhibitory activity than the standard. Among the selected flavonoids, butein, biochanin and herbacetin showed excellent binding interactions with aldose reductase enzyme than the standard. This may be attributed due to the differences in the position of the functional groups in the compounds.
Table IV: Intermolecular energies of the compounds based on their rank
Compound | Intermolecular energies of the compounds based on their rank (kcal/mol) | |||||||||
---|---|---|---|---|---|---|---|---|---|---|
1 | 2 | 3 | 4 | 5 | 6 | 7 | 8 | 9 | 10 | |
Biochanin | -10.1 | -10.1 | -10.1 | -10.1 | -10.1 | -10.1 | -10.0 | -9.9 | -9.0 | -9.0 |
Butein | -11.4 | -11.0 | -10.9 | -10.6 | -10.5 | -10.0 | -11.1 | -10.2 | -9.4 | -9.1 |
Esculatin | -7.8 | -7.8 | -7.8 | -7.8 | -7.8 | -7.8 | -7.8 | -7.8 | -7.7 | -7.7 |
Fisetin | -10.2 | -10.2 | -10.1 | -10.0 | -9.6 | -9.6 | -10.2 | -9.6 | -9.5 | -9.9 |
Herbacetin | -10.6 | -9.4 | -8.4 | -8.4 | -8.3 | -8.3 | -8.3 | -8.2 | -8.2 | -7.9 |
Epalrestat | -10.5 | -10.4 | -10.4 | -9.9 | -9.6 | -9.6 | -9.4 | -9.0 | -9.5 | -8.7 |
Conclusion
These results clearly indicate that the flavonoids especially, butein, biochanin and herbacetin showed excellent binding interactions with aldose reductase enzyme than the standard. This in silico studies is actually an added advantage to screen the aldose reductase enzyme inhibition.
References
Azam F, Prasad MVV, Thangavel N, Ali HI. Molecular docking studies of 1-(substituted phenyl)-3-(naphtha [1,2–d] thiazol-2-yl) urea/thiourea derivatives with human adenosine A2A receptor. Bioinformation 2011; 6: 330-34.
Cushnie TP, Lamb AJ. Recent advances in understanding the antibacterial properties of flavonoids. Int J Antimicrob Agents. 2011; 38: 99-107.
Dong Y, Yang J, Ren X, Zhang H, He J. New Aldose Reductase Inhibitors N99-596 A and B from Streptomyces. J Antibiot. 2005; 58: 737-39.
González R, Ballester I, López-Posadas R, Suárez MD, Zarzuelo A, MartÃnez-Augustin O, Sánchez de Medina F. Effects of flavonoids and other polyphenols on inflammation. Crit Rev Food Sci Nutr. 2011; 51: 331-62.
Guzman A, Guerrero RO. Inhibition of aldose reductase by herbs extracts and natural substances and their role in prevention of cataracts. Rev Cubana Plant Med. 2005; 10: 3-4.
Hwang YC, Shaw S, Kaneko M, Redd H, Marrero MB, Ramasamy R. Aldose reductase pathway mediates JAK-STAT signaling: A novel axis in myocardial ischemic injury. FASEB J. 2005; 19: 795-97.
Lamba HS, Bhargava CS, Thakur M, Bhargava S. αÂGlucosidase and aldose reductase inhibitory activity in vitro and antiÂdiabetic activity in vivo of tribulus terrestris L. (dunal). Int J Pharm Pharmaceut Sci. 2011; 3: 270-72.
Liu HB, Wang ZL, Qiao YX, Zhou JJ. Flavonoids with aldose reductase inhibiting activity: Pharmacophore modeling and implications for mechanism. Acta Phys-Chim Sin. 2007; 23: 1059-64.
Madeswaran A, Umamaheswari M, Asokkumar K, Sivashanmugam T, Subhadradevi V, Jagannath P. In silico docking studies of lipoxygenase inhibitory activity of commercially available flavonoids. J Comput Methods Mol Des. 2011; 1: 65-72.
Madeswaran A, Umamaheswari M, Asokkumar K, Sivashanmugam T, Subhadradevi V, Jagannath P. Docking studies: In silico phosphodiesterase inhibitory activity of commercially available flavonoids. Bangladesh J Pharmacol. 2012; 7: 70-75.
Nishiumi S, Miyamoto S, Kawabata K, Ohnishi K, Mukai R, Murakami A, Ashida H, Terao J. Dietary flavonoids as cancer-preventive and therapeutic biofactors. Front Biosci. 2011; 3: 1332-62.
Norgan AP, Coffman PK, Kocher JPA, Katzmann DJ, Sosa CP. Multilevel parallelization of AutoDock 4.2. J Cheminform. 2011; 3: 12.
Ravindranath TM, Mong PY, Ananthakrishnan R, Li Q, Quadri N, Schmidt AM, Ramasamy R, Wang Q. Novel role for aldose reductase in mediating acute inflammatory responses in the lung. J Immunol. 2009; 183: 8128-37.
Saraswat M, Muthenna P, Suryanarayana P, Petrash JM, Reddy GB. Dietary sources of aldose reductase inhibitors: Prospects for alleviating diabetic complications. Asia Pac J Clin Nutr. 2008; 17: 558-65.
Seeliger D, de Groot BL. Ligand docking and binding site analysis with PyMOL and Autodock/Vina. J Comput Aid Mol Des. 2010; 24: 417-22.
Umamaheswari M, Madeswaran A, Asokkumar K, Sivashanmugam AT, Subhadradevi V, Jagannath P. Docking studies: Search for possible phytoconstituents for the treatment of gout. Int J Biol Pharm Res. 2012; 3: 6-11.
Umamaheswari M, Madeswaran A, Asokkumar K, Sivashanmugam T, Subhadradevi V, Jagannath P. Study of potential xanthine oxidase inhibitors: In silico and in vitro biological activity biological activity biological activity biological activity. Bangladesh J Pharmacol. 2011; 6: 117-23.
Vianna R, Brault A, Martineau LC, Couture R. In vivo anti-diabetic activity of the ethanolic crude extract of sorbus decora c.k.schneid. (rosacea): A medicinal plant used by canadian james bay cree nations to treat symptoms related to diabetes. Inventi Impact Ethno Pharmacol. 2011; 3: 10-15.
Yadav UCS, Naura AS, Aguilera-Aguirre L, Ramana KV, Boldogh I, Sur S, Boulares HA, Srivastava SK. Aldose reductase inhibition suppresses the expression of Th2 cytokines and airway inflammation in ovalbumin-induced asthma in mice. J Immunol. 2009; 183: 4723-32.
Zhang S, Kumar K, Jiang X, Wallqvist A, Reifman J. DOVIS: An implementation for high throughput virtual screening using AutoDock. BMC Bioinformatics 2008; 9: 126.
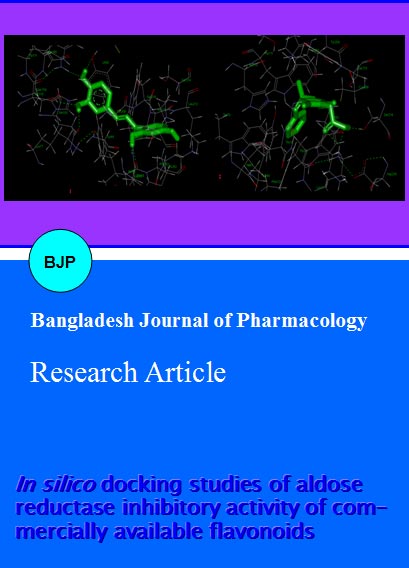