In silico prediction of functional loss of cst3 gene in hereditary cerebral amyloid angiopathy
Abstract
The computational identification of missense mutation in CST3 (CYSTATIN 3 or CYSTATIN C) gene has been done in the present study. The missense mutations in the CST3 gene will leads to hereditary cerebral amyloid angiopathy The initiation of the analysis was done with SIFT followed by POLY-PHEN-2 and I-Mutant 2.0 using 24 variants of CST3 gene of Homo sapiens which were derived from dbSNP. The analysis showed that 5 variants (Y60C, C123Y, L19P, Y88C, L94Q) were found to be less stable and damaging by SIFT, POLYPHEN-2 and I-MUTANT2.0. Furthermore the outputs of SNP & GO are collaborated with PHD-SNP (Predictor of Human Deleterious-Single Nucleotide Polymorphism) and PANTHER to predict 5 variants (Y60C, Y88C, C123Y, L19P, and L94Q) having clinical impact in causing the disease. These findings will be certainly helpful for the present medical practitioners for the treatment of cerebral amyloid angiopathy.
Introduction
Single nucleotide polymorphisms (SNPs) are the most abundant form of genetics variations in the human genome. Most of the SNPs in the human genome are present in the non-coding DNA consisting of 5' and 3' and translated regions (UTR) (Rajasekaran et al., 2007). The dbSNP is used for the same and it is a public domain archive (Sherry et al., 2001).
The gene, CST3, codes for human cystatin C, and has the same organization as the CST1 gene for cystatin SN and the CST2 gene for cystatin SA (Saitoh et al., 1989). It has been found to play a role in brain disorder example amyloid (a specific type of protein deposition) (Goate et al., 1991).
Analysis found that missense mutation in the CST3 gene lead to a condition called hereditary cerebral amyloid angiopathy. This condition is characterised by stock and dementia which begins in mid adulthood. CST3 gene is located from base pair 23, 608, 533 to base pair 23, 618, 684 on chromosome 20 (Saitoh et al., 1989). As far as presence scenario is concerned the discovery of deleterious SNPs is crucial task for pharmacogenomics and pharmacogenetics. We undertook this work basically to perform a computational analysis of CST3 gene consisting of ns SNPs and identification of possible deleterious mutation. Out of the 24 SNPs, the most deleterious SNPs which are significant in causing disease are Y60C, C123Y, L19P, Y88C, and L94Q. These mutations can be a candidate of most concern in the disease hereditary cerebral amyloid angiopathy caused by CST3 gene.
Materials and Methods
Dataset
db-SNP (http://www.ncbi.nlm.nih.gov/SNP/) is used to obtain the SNPs and their related protein sequence for CST3 gene of Homo sapiens for the computational analysis. (Arnold et al., 2006). Every SNP consist of an unique ID, reference ID (rsIDs). Complete information about that SNP as well as the amino acid changes, their respective positions and corresponding accessions IDs are obtained by clicking on each rsIDs. Clicking on accessions ID delivers information regarding the protein encoded by the genes. Also we are thankful for the availability of numerous comprehensive and easy to use software packages and web-based services to detect the structures (Kumar et al., 2009).
Sequence homology based method (SIFT)-Analysis of functional effect of point's mutations
The damaging single amino acid polymorphism detected by the SIFT programme (Ng and Henikoff, 2003). The main concept behind this technique is mainly based on the evolutionary amino acid conservation with in protein families. The more the conserved positions are the more they are intolerant to substitution where as the vice versa is also true. Therefore, the results are deleterious or damaging when the changes occurs at well conserved positions. Protein sequence forms of queries are being submitted. SIFT works by using multiple sequence alignment information on a considered query sequence for the prediction (Capriotti et al., 2005) of tolerated as well as deleterious substitution for each position for the query sequence. The multistep SIFT process consist of a) protein database search for related sequences, b) sequence alignment build-up, c) probability scaling at every position from the alignment. The cut off value of tolerance index for SIFT program >0.5. the tolerance index is inversely proportions to the impact of amino acid substitutions that is higher the tolerance index lesser the impact of substitution and lesser the tolerance index the higher the functional impact of amino acid substitution.
Structure and sequence based method-POLYPHEN2 (polymorphism phenotyping v2)
POLYPHEN2 is a physical and comparison based tool that shows the impact of amino acid substitution on the structure and function of human protein (Ramensky et al., 2002). The input is a protein sequence with mutational positions and two variants of amino acids. This is followed by PSIC scores calculations for both the variants and then the difference between two are computed. The greater the PSIC score difference the higher the functional impact of particular amino acid substitution.
Stability analysis- I-Mutant 2.0
It is a SVM based tools that is i.e, which is support vector machine based tool. I-Mutant2.0 leads to automatic protein stability change prediction which is caused by single point mutation (Capriotti et al., 2005). The initiations were done either by using protein structure or more precisely from the protein sequence. The output is a free energy change value (ΔΔG). Positive ΔΔG value infers that the protein being mutated is of higher stability and vice versa is also true.
SNPs & GO- (disease related mutations predictions)
SNPs tends for Single Nucleotide Polymorphism data base and GO is Gene Ontology. Like I-Mutant2.0 SNPs & GO (Calabrese et al., 2009) is also a support vector machine (SVM) which is based on the method to accurately predict the mutation related to disease from protein sequence. The input is the FASTA sequence of the whole protein, the output is based on the difference among the neutral and disease related variations of the protein sequence. The RI (reliability index) with value of greater than 5 depicts the disease related effect caused by mutation on the function of parent protein. The PHD SNP (Altschul et al., 1997) & PANTHER algorithms were also used in the display of output.
Result and Discussion
There are 24 missense mutation were found namely Y60C, P33L, V75M, V44M, M67K, A129T, Y88C, A72S, D113A, A2S, G30S, T98M, C123Y, T142A, L19P,L94Q, R71S, R71H, V17M, G3R, R96G, G38A, R79H, A25T. These mutations were retrieved from dbSNP (Smigielski et al., 2000).
The mutations were one by one submitted in SIFT program for the tolerance index (Ng and Henikoff, 2003) check. Out of the 24 variants, 8 variants were found to be deleterious with a tolerance index score of >.05. The result has been depicted in Table I. It was observed that 4 out of 8 variants were highly deleterious with a tolerance index score of 0. One variant with a tolerance index of 0.01, one with 0.03, one with 0.04 and one with 0.05.
The POLYPHEN2 program (Ramensky et al., 2002) was used after SIFT with protein sequence having mutational position submitted as inputs, A PSIC score > 0.950 were found to be probably damaging, A PSIC score of >0.5 were found to be possibly damaging and the rest were found to be benign (Table I).
Table I: List of nsSNP predicted as deleterious, damaging and less stable by SIFT, PolyPhen-2 and I-Mutant respectively
rsID | AA change | Tolerance index | PSIC SD | Prediction | Stability |
---|---|---|---|---|---|
rs377450166 | Y60C | 0.03 | 0.999 | Probably damaging | Decrease |
rs375692362 | P33L | 0.32 | 0.051 | Benign | Decrease |
rs373743268 | V75M | 0 | 1 | Probably damaging | Increase |
rs373213120 | V44M | 0.16 | 0.867 | Possibly damaging | Decrease |
rs373177867 | M67K | 0.92 | 0 | Benign | Decrease |
rs371605207 | A129T | 0.63 | 0.01 | Benign | Decrease |
rs371124032 | Y88C | 0 | 1 | Probably damaging | Decrease |
rs202145575 | A72S | 0.09 | 0.37 | Benign | Decrease |
rs201184716 | D113A | 0.38 | 0.607 | Possibly damaging | Decrease |
rs200984369 | A2S | 0 | 0.939 | Possibly damaging | Decrease |
rs200245337 | G30S | 0.72 | 0.01 | Benign | Decrease |
rs200037041 | T98M | 0.09 | 0.975 | Probably damaging | Decrease |
rs149051742 | C123Y | 0.05 | 1 | Probably damaging | Decrease |
rs141643699 | T142A | 0.45 | 0.002 | Benign | Decrease |
rs113550984 | L19P | 0.01 | 0.94 | Possibly damaging | Increase |
rs28939068 | L94Q | 0 | 0.988 | Probably damaging | Decrease |
rs11542364 | R71S | 0.44 | 1 | Probably damaging | Decrease |
rs11542360 | R71H | 0.1 | 0.999 | Probably damaging | Decrease |
rs11542359 | V17M | 0.1 | 0.63 | Possibly damaging | Decrease |
rs11542357 | G3R | 0.09 | 0.002 | Benign | Decrease |
rs11542355 | R96G | 0.16 | 1 | Probably damaging | Decrease |
rs11542354 | G38A | 0.2 | 0.243 | Benign | Decrease |
rs11542353 | R79H | 0.04 | 0.999 | Probably damaging | Decrease |
rs1064039 | A25T | 0.41 | 0.003 | Benign | Decrease |
Following the POLYPHEN2 was I-Mutant2 program for the analysis. The program tells about protein structure stability, out of 24 variants 22 variants were found to have less stability (Table I). The transformations that happened in the amino acids as a result of the missense mutations are Y60C (polar amino acid to a polar amino acid), P33L (non-polar amino acid to non-polar amino acid), V75M (non-polar amino acid to non-polar amino acid), V44M (non-polar amino acid to non-polar amino acid), M67K (non-polar amino acid to polar basic amino acid), A129T (non-polar amino acid to polar amino acid), Y88C (polar amino acid to polar amino acid), A72S (non-polar amino acid to polar amino acid), D113A (polar acidic amino acid to non-polar amino acid), A2S (non-polar amino acid to polar amino acid), G30S (non-polar amino acid to polar amino acid), T98M(polar amino acid to non-polar amino acid), C123Y (polar amino acid to polar amino acid), T142A (polar amino acid to non-polar amino acid), L19P (non-polar amino acid to non-polar amino acid), L94Q (non-polar amino acid to polar amino acid), R71S (polar basic amino acid to polar amino acid), R71H (polar basic amino acid to polar basic amino acid), V17M (non-polar amino acid to non-polar amino acid), G3R (non-polar amino acid to polar basic amino acid), R96G (polar basic amino acid to non-polar amino acid), G38A (non-polar amino acid to non-polar amino acid), R79H (polar basic amino acid to polar basic amino acid), A25T (non-polar amino acid to polar amino acid). It can be said that by preserving the pysico chemical properties of amino acids may not necessarily result in mutations that are harmless.
Out of the 24 variants, 8 variants namely Y60C, C123Y, L19P, R79H, V75M, Y88C, A2S, L94Q were found to be deleterious and damaging by all the three programs that is SIFT, POLPHEN 2 and I-Mutant2.0 (Capriotti et al., 2005). The SNPs and GO server predicted 7 variants as disease causing mutation (Table II), whereas PHD-SNP server predicted 12 variants to be disease related (Table III), and PANTHER predicted 11 variants as disease (Table IV). Finally combining the results of all the programs, 5 variants namely Y60C, Y88C, C123Y, L19P and L94Q were predicted to have functional effect on protein function and stability (Table V), and further these functionally significant variants were superimposed with native structure using PyMol (Figure 1).
Table II: List of nsSNP predicted as disease associated by SNP & GO server
rsID | AA change | SNP & GO prediction | Probability score | RI |
---|---|---|---|---|
rs377450166 | Y60C | disease | 0.73 | 5 |
rs375692362 | P33L | neutral | 0.048 | 9 |
rs373743268 | V75M | neutral | 0.416 | 2 |
rs373213120 | V44M | neutral | 0.021 | 10 |
rs373177867 | M67K | neutral | 0.145 | 7 |
rs371605207 | A129T | neutral | 0.072 | 9 |
rs371124032 | Y88C | disease | 0.835 | 7 |
rs202145575 | A72S | neutral | 0.107 | 8 |
rs201184716 | D113A | neutral | 0.033 | 9 |
rs200984369 | A2S | neutral | 0.015 | 10 |
rs200245337 | G30S | neutral | 0.037 | 9 |
rs200037041 | T98M | neutral | 0.056 | 9 |
rs149051742 | C123Y | disease | 0.9 | 8 |
rs141643699 | T142A | neutral | 0.012 | 10 |
rs113550984 | L19P | disease | 0.537 | 1 |
rs28939068 | L94Q | disease | 0.682 | 4 |
rs11542364 | R71S | disease | 0.56 | 1 |
rs11542360 | R71H | neutral | 0.357 | 3 |
rs11542359 | V17M | neutral | 0.054 | 9 |
rs11542357 | G3R | neutral | 0.009 | 10 |
rs11542355 | R96G | disease | 0.541 | 1 |
rs11542354 | G38A | neutral | 0.034 | 9 |
rs11542353 | R79H | neutral | 0.253 | 5 |
rs1064039 | A25T | neutral | 0.042 | 9 |
Table III: List of nsSNP predicted as disease associated by PHD-SNP server
rsID | AA change | PHD-SNP prediction | Probability score | RI |
---|---|---|---|---|
rs377450166 | Y60C | Disease | 0.962 | 9 |
rs375692362 | P33L | Neutral | 0.195 | 6 |
rs373743268 | V75M | Disease | 0.863 | 7 |
rs373213120 | V44M | Neutral | 0.148 | 7 |
rs373177867 | M67K | Disease | 0.625 | 3 |
rs371605207 | A129T | Neutral | 0.348 | 3 |
rs371124032 | Y88C | Disease | 0.991 | 10 |
rs202145575 | A72S | Disease | 0.509 | 0 |
rs201184716 | D113A | Neutral | 0.324 | 4 |
rs200984369 | A2S | Neutral | 0.132 | 7 |
rs200245337 | G30S | Neutral | 0.341 | 3 |
rs200037041 | T98M | Neutral | 0.499 | 0 |
rs149051742 | C123Y | Disease | 0.993 | 10 |
rs141643699 | T142A | Neutral | 0.03 | 9 |
rs113550984 | L19P | Disease | 0.954 | 9 |
rs28939068 | L94Q | Disease | 0.897 | 8 |
rs11542364 | R71S | Disease | 0.889 | 8 |
rs11542360 | R71H | Disease | 0.824 | 6 |
rs11542359 | V17M | Neutral | 0.427 | 1 |
rs11542357 | G3R | Neutral | 0.044 | 9 |
rs11542355 | R96G | Disease | 0.931 | 9 |
rs11542354 | G38A | Neutral | 0.365 | 3 |
rs11542353 | R79H | Disease | 0.718 | 4 |
rs1064039 | A25T | Neutral | 0.254 | 5 |
Table IV: List of nsSNP predicted as disease associated by PANTHER server
rsID | AA change | PANTHER prediction | Probability score | RI |
---|---|---|---|---|
rs377450166 | Y60C | Disease | 0.975 | 10 |
rs375692362 | P33L | Disease | 0.504 | 0 |
rs373743268 | V75M | Disease | 0.808 | 6 |
rs373213120 | V44M | Neutral | 0.294 | 4 |
rs373177867 | M67K | Neutral | 0.371 | 3 |
rs371605207 | A129T | Neutral | 0.391 | 2 |
rs371124032 | Y88C | Disease | 0.973 | 9 |
rs202145575 | A72S | Neutral | 0.365 | 3 |
rs201184716 | D113A | Neutral | 0.188 | 6 |
rs200984369 | A2S | Neutral | 0.038 | 9 |
rs200245337 | G30S | Neutral | 0.112 | 8 |
rs200037041 | T98M | Neutral | 0.392 | 2 |
rs149051742 | C123Y | Disease | 0.995 | 10 |
rs141643699 | T142A | Neutral | 0.189 | 6 |
rs113550984 | L19P | Disease | 0.734 | 5 |
rs28939068 | L94Q | Disease | 0.859 | 7 |
rs11542364 | R71S | Disease | 0.817 | 6 |
rs11542360 | R71H | Disease | 0.848 | 7 |
rs11542359 | V17M | Neutral | 0.352 | 3 |
rs11542357 | G3R | Neutral | 0.099 | 8 |
rs11542355 | R96G | Disease | 0.847 | 7 |
rs11542354 | G38A | Neutral | 0.23 | 5 |
rs11542353 | R79H | Disease | 0.628 | 3 |
rs1064039 | A25T | Neutral | 0.294 | 4 |
Table V: List of nsSNP predicted as disease associated by SNP & GO, PHD-SNP and PANTHER server
rsID | AA change | SNP & GO | PHD-SNP | PANTHER |
---|---|---|---|---|
rs377450166 | Y60C | Disease | Disease | Disease |
rs149051742 | C123Y | Disease | Disease | Disease |
rs113550984 | L19P | Disease | Disease | Disease |
rs371124032 | Y88C | Disease | Disease | Disease |
rs28939068 | L94Q | Disease | Disease | Disease |
Figure 1: Superimposed view of C60Y (A), Y88C (B), C123Y(C), L19P (D) and L94Q (E) rendered using PyMol
Conclusion
We examined clinically important mutations in CST3 gene by means of different genomic algorithms. We certainly believe that this analysis will have immense importance in clinical management of cerebral amyloid angiopathy.
Acknowledgement
The authors would like to thank management of VIT University for providing the facilities to carry out this work.
References
Altschul SF, Madden TL, Schäffer AA, Zhang J, Zhang Z, Miller W, Lipman DJ. Gapped BLAST and PSI-BLAST: A new generation of protein database search programs. Nucleic Acids Res. 1997; 25: 3389-402.
Arnold K, Bordoli L, Kopp J, Schwede T. The SWISS-MODEL Workspace: A web-based environment for protein structure homology modeling. Bioinformatics 2006; 22: 195-201.
Calabrese R, Capriotti E, Fariselli P, Martelli PL, Casadio R. Functional annotations improve the predictive score of human disease-related mutations in proteins. Hum Mutat. 2009; 30: 1237-44.
Capriotti E, Fariselli P, Casadio R. I-Mutant2.0: Predicting stability changes upon mutation from the protein sequence or structure. Nucleic Acids Res. 2005; 33: W306-10.
Goate A, Chartier-Harlin MC, Mullan M, Brown J, Crawford F, Fidani L, Giuffra L, Haynes A, Irving N, James L, Mant R, Newton P, Rooke K, Roques P, Talbot C, Pericak-Vance M, Roses A, Williamson R, Rossor M, Owen M, Hardy J. Segregation of a missense mutation in the amyloid precursor protein gene with familial Alzheimer's disease. Nature 1991; 349: 704-06.
Johansson MU, Zoete V, Michielin O, Guex N. Defining and searching for structural motifs using DeepView/Swiss-PdbViewer. BMC Bioinformatics. 2012; 13: 173.
Kumar P, Henikoff S, Ng PC. Predicting the effects of coding non- synonymous variants on protein function using the SIFT algorithm. Nat Protoc. 2009; 4: 1073-81.
Ng PC, Henikoff S. Predicting deleterious amino acid substitutions. Genome Res. 2001; 11: 863-74.
Ng PC, Henikoff S. SIFT: Predicting amino acid changes that affect protein function. Nucleic Acids Res. 2003; 31: 3812-14.
Rajasekaran R, Sudandiradoss C, Doss CG, Sethumadhavan R. Identification and in silico analysis of functional SNPs of the BRCA1 gene. Genomics 2007; 90: 447-52.
Ramensky V, Bork P, Sunyaev S. Human non-synonymous SNPs: Server and survey. Nucleic Acids Res. 2002; 30: 3894-900.
Saitoh E, Sabatini LM, Eddy RL, Shows TB, Azen EA, Isemura S, Sanada K. The human cystatin C gene (CST3) is a member of the cystatin gene family which is localized on chromosome 20. Biochem Biophys Res Commun. 1989; 162: 1324-31.
Sherry ST, Ward MH, Kholodov M, Baker J, Phan L, Smigielski EM, Sirotkin K. dbSNP: The NCBI database of genetic variation. Nucleic Acids Res. 2001; 29: 308-11.
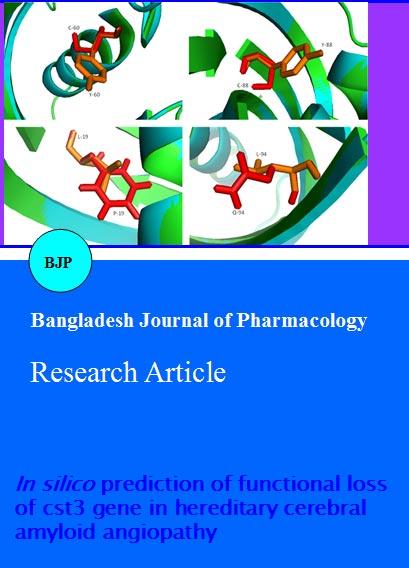