A computational approach to analyze the missense mutations in human angiogenin variants leading to amyotrophic lateral sclerosis
Abstract
The most deleterious missense mutations of angiogenin forming amyotrophic lateral sclerosis were identified computationally and the substrate binding efficiencies of these mutations were also analyzed. Out of 12 variants, I-Mutant2.0, SIFT and PolyPhen programs identified 3 variants that were less stable, deleterious and damaging respectively. These 3 variants were modeled to find their conformational changes in concern with native angiogenin through RMSD calculation and Total energy. Docking of native and 3 mutants with ribonuclease inhibitor was performed to describe the binding efficiencies of those detrimental missense mutations. By computing the binding amino acids’ flexibility of angiogenin and computing the binding free energy (ΔG) between native and mutant complexes, the loss in binding affinity with their interacting protein namely ribonuclease inhibitor was investigated.
Introduction
Amyotrophic lateral sclerosis (ALS) is a fatal neurode-generative disorder characterized by selective destruction of motor neurons, resulting paralysis in stages and death within a few years from onset (Bruijin et al., 2004). In the past decade, a small number of genes involved in the etiology of the disease have been identified. Angiogenin (ANG), which encodes an angiogenic protein, has been recently identified as a candidate susceptibility gene for ALS in the Irish and Scottish population by Greenway et al (Greenway et al., 2004).
The ANG gene provides instructions for making a protein called angiogenin. This protein promotes the formation of new blood vessels from pre-existing blood vessels through a process called angiogenesis. The ANG gene is located on the long (q) arm of chromosome 14 between positions 11.1 and 11.2. More precisely, the ANG gene is located from base pair 21,152,335 to base pair 21,162,344 on chromosome 14. The protein aggregation, defective axonal transport, oxidative stress, dysfunctional growth factor signaling, mitochondrial dysfunction and excitotoxicity are some of the factors found to be combined to the inception of ALS.
Around 10% of ALS cases are inherited genetically, but the other 90% have no clear genetic cause (Dion et al., 2009). Many ALS-linked genes have been identified, including SOD1 (encoding superoxide dismutase 1), TARDBP (encoding TAR DNA binding protein-43), FUS/TLS (encoding RNA-binding protein FUS), and others (Vande Velde et al., 2011). Among other genes, heterozygous missense mutations in ANG have been associated with ALS (Greenway et al.). Mature ANG is a 14 kDa, 123 amino acid, secreted protein primarily produced by the liver (Kishikawa et al., 2008). ANG is broadly expressed throughout the adult human nervous system and is neuroprotective (Kieran et al., 2008; Kishikawa et al., 2008). Further, in ALS patients, the identified ANG missense variants cause the reduced angiogenic activity of ANG in endothelial cells (Wu et al., 2007) and impairment of neurite extension and survival of motor neurons (Kieran et al., 2008; Subramanian et al., 2008). ANG parameterized three characteristic features: (i) the characteristic ribonuclease activity towards poly-, di- and cyclic nucleotides is 104-106 lower and also varied enzymatic specificity (Shapiro et al., 1986) (ii) the region between residues 58–70 seems to constitute a 'cell-binding site', independent from the active site (Hallahan et al., 1991, 1992) perhaps involved essentially in protein-protein interactions; and (iii) the region 31-35 contributes a nucleolar localization signal (Moroianu et al., 1994).
A large (~450 residues, ~49 kDa), acidic (pI ~4.7) protein referred to as Ribonuclease inhibitor (RI) which has leucine-rich repeat is tightly complexed to some ribonucleases. Also being an important cellular protein, it consists ~0.1% of all cellular protein by weight, and found to regulate the RNA lifetime (Shapiro, 2001). Possibly, for any protein-protein interaction, the affinity of RI for ribonucleases found to be the highest; the dissociation constant of the RI-Rnase. A complex is around 20 fM under physiological conditions whereas it is even smaller (<1 fM) for the RI-angiogenin complex. Eminently, RI could bind a huge variety of RNases, in spite of its low sequence identity. RNases bind as a "cork in the bottle" as indicated by structural studies, associating primarily with the C-terminal end of RI; the interaction is highly electrostatic however occupies a huge surface area (>25 nm2). The cytotoxic and cytostatic effects of Ribonuclease made it to examine as potential cancer therapeutics. Hence RI's affinity for ribonucleases is important (Kobe et al., 1996). Recent experimental studies suggest that the loss of either nuclear translocation activity, ribonucleolytic activity or both leads to total loss of angiogenin function due to mutations in ANG which leads to ALS (Wu et al., 2007). Above 14 ANG mutations found to be linked with ALS wherein 10 have been analyzed intensively, whereas the functional loss of ANG caused by these mutations and the cause of its molecular mechanism is unclear (Padhi et al., 2012).
Materials and Methods
Datasets: The data on the protein sequence and its variants (single amino acid polymorphisms/missense mutations/point mutations) of ANG were retrieved from the Swissprot database available at http://www.expasy.ch/sprot/. The segments of every Swissprot entry imparted details on polymorphic variants, some of which were disease(s) - associated by causing defects in a given protein (Yip et al., 2004, 2008; Boeckmann et al., 2003). The 3D structure of angiogenin and their complexes were extracted from Protein Data Bank with PDB ID 2ANG (Berman et al., 2000) for mutant modeling and docking studies according to harmful point mutants.
Prediction of stability changes caused by SAPs using Support Vector Machine (I-Mutant2.0): We used the program I-Mutant2.0 available at http://gpcr.biocomp. unibo.it/cgi/predictors/IMutant2.0/ I-Mutant2.0. cgi. I-Mutant2.0, a support vector machine (SMV) based tool worked out the automatic prediction of protein stability change upon single amino acid substitution. Starting either from the protein structure or from the protein sequence, the predictions were done through I-Mutant2.0 (Capriotti et al., 2005). Here, a data set used derived from ProTherm (Bava et al., 2004) is the database considered when compared against others for thermodynamic experimental data of free energy changes concerning protein stability caused by mutations in various environment. The output files indicated the predicted free energy change value or sign (∆∆G), which was based on the calculation, the unfolding Gibbs free energy value of the mutated protein minus the unfolding Gibbs free energy value of the native protein (kcal/mol). Positive ∆∆G values indicated high stability for mutated protein and negative values meant a low stability.
Analysis of functional effects of SAPs by a Sequence Homology-Based Method (SIFT): The program SIFT (Ng et al., 2003) available at http://blocks.fhcrc.org/sift/SIFT.html was employed, which specifically gives an unprecedented level of control required to identify deleterious single amino acid polymorphisms. SIFT, a sequence homology-based tool postulates the conservation of essential amino acids in a family of protein; therefore, mutations at well-conserved locales have a tendency to be deleterious (Ng et al., 2001). Queries are submitted in the sequence format for proteins. Once the query sequences are loaded in SIFT, they can be used to generate a multiple sequence alignment from which the tolerated and deleterious substitutions are predicted for every position of the query sequence. Substitutions at each position with normalized probabilities less than a chosen cutoff were predicted to be deleterious and those greater than or equal to the cutoff are predicted to be tolerated (Ng et al., 2003). The cutoff value in SIFT program was tolerance index of ≥0.05. The higher the tolerance index, the less functional impact a particular amino acid substitution would be likely to have.
Simulation for functional effects in SAPs by structure homology based method (PolyPhen): Analyzing the damage caused by point mutations at the structural level was considered very important to understand the functional activity of the protein. In order to focus this issue, we used the server PolyPhen (Ramensky et al., 2002), available at http://coot.embl.de/PolyPhen/. We parameterized the protein sequence, SWALL database ID or accession number, together with the sequence position of two amino acid variants as input options for the PolyPhen server. The query was submitted in the sequence format for a protein with a mutational position and two amino acid variants. In order to calculate the score, PolyPhen server employed the sequence-based characterization of the substitution site, profile analysis of homologous sequences, and mapping of the substitution site to known protein 3D structures. The position-specific independent counts (PSIC) scores were calculated for every two variants, followed by computing the PSIC score variation amid them. The higher the PSIC score difference, the greater being the functional impact of a particular amino acid substitution.
Computing total energy and RMSD by modeling SAP location on protein 3D Structure: In order to compute the total energy and to evaluate the structural divergence between the native type and mutant types by RMSD (Root Mean square Deviation), the structural analysis was executed. Protein Data Bank (15) and Single Amino Acid Polymorphism database (SAAPdb) (Cavallo et al., 2005) were employed for finding the Angiogenin protein (PDB ID: 2ANG) 3D structure. Also the position of mutation and the mutated residue in PDB ID 2ANG was checked out. SWISSPDB viewer brought about the mutation and NOMAD-Ref server executed the energy minimization for 3D structures (Lindahl et al., 2006). Depending on the system of steepest descent, conjugate gradient and L-BFGS (Delarue et al., 2004), this server was carried out with Gromacs as default force field for energy minimization. To minimize the energy of the 3D structure of angiogenin, the conjugate gradient method was employed. Also we employed the ifold server (Sharma et al., 2006) to optimize the angiogenin 3D structure, for simulated annealing, which depend on distinct molecular dynamics. This server efficiently represents the immense conformational space of biomolecules based on both the length and time scales. Substitutions, deletions and insertions could be the major cause for the divergence of the mutant structure from the native structure (Han et al., 2006) and also these two structures diverge to modify the functional activity (Varfolomeev et al., 2002) concerned with the inhibitors’ binding efficiency as assessed through RMSD calculation.
Computation of intramolecular interactions by PIC server: In order to compute the intramolecular interactions for both the native and mutant structures, PIC server was utilized. PIC (Protein Interactions Calculator) server acquires the caretesian coordinates of protein structure in the PDB format. In perceiving the molecular basis of stability and proteins’ function and their complexes, the inter protein structure interactions and the intra protein interactions in an congregation were considered important. It emphasized several weak and strong interactions which includes interaction between apolar residues, disulphide bridges, hydrogen bonds influencing secondary and tertiary structure of protein, ionic (electrostatic) interactions, aromatic-sulphur interactions, cation-π interactions and aromatic-aromatic interactions. The PIC server (Tina et al., 2007) has been available at: http://crick.mbu.iisc.ernet.in/∼PIC.
Analyzing the binding affinity between angiogenin and ribonuclease inhibitor: For finding the binding affinity between ANG and RI (Ribonuclease inhibitor) complex, we used the protein-protein docking server, GRAMM-X (http://vakser.bioinformatics.ku.edu/resources/gramm/grammx), GRAMM Fast Fourier Transforma tion methodology based tool by employing smoothed potentials, refinement stage, and knowledge-based scoring. It represented a new implementation that uses a smoothed Lennard-Jones potential on a fine grid during the global search FFT stage, followed by the refinement optimization in continuous coordinates and rescoring with several knowledge-based potential terms (Tovchigrechiko et al., 2006). Then, the docked protein complex was given to the DFIRE server as an input for calculating the binding free energy (ΔG) score. It used a new reference state called the distance-scaled, finite ideal-gas reference (DFIRE) state. It was a distance-dependent structure-derived potential developed so far and all employed a reference state that could be characterized as a residue (atom) -averaged state. In addition, the DFIRE-based all-atom potential provided the most accurate prediction of the stabilities of mutants based on knowledge-based all-atom potentials.
Depicting the binding pocket flexibility using normal mode analysis: The atomic motions in proteins measured quantitatively could be acquired from the mean square fluctuations of the atoms corresponding to their regular positions. This could be correlated to the B-factor (Yuan et al., 2005; Ringe et al., 1986). Therefore, the study of B-factors provided insights into protein motions, the flexibility of amino acids and protein stability (Parthasarathy et al., 2000). In addition, the protein function and various types of interactions depends on protein flexibility of certain amino acids in a protein (Carlson et al., 2000). The key parameter for understanding the binding efficiency was the flexibility of amino acids in the binding pocket. Indeed, the binding effect was impaired by the loss of flexibility (Hinkle et al., 2003) and vice versa. So, the Bfactor helped in analyzing this, which is computed from the mean-square displacement <R2> of the lowest frequency normal mode using the ElNemo server (Suhre et al., 2004).
Result and Discussion
The 2ANG and a total of 12 variants namely Q36L, K41E, K41I, S52N, R55K, C63W, K64I, I70V, K84E, P136L, V137I and H138R analyzed in our study were extracted from Swissprot database.
Among the 12 variants, 10 variants were shown less stable using the I-Mutant2.0 server (16) (Table I). Among these 10 variants, three variants showed a ΔΔG value <-2.0, two variants showed a ΔΔG value <-1.0 and five variants showed a ΔΔG value >-1.0 as in (Table I). Among the 10 variants that indicated a negative ΔΔG, two variants (K41E and K84E) altered their positively charged amino acid into negatively charged amino acid, one variant (S52N) retained its polar uncharged property. One variant, (R55K) retained its positively charged property and two variants (I70V and V137I) retained its non polar properties followed by variants viz., Q36L, K41I, C63W and H138R which changed amino acids from polar uncharged to non polar, positively charged to non polar, polar uncharged into aromatic and positive into aromatic. Infact, only in the view of amino acid substitution concerned with physicochemical properties, the detrimental effect might not perhaps be identified. Instead, taking account of the sequence conservation in addition to the above believed properties could be advantageous and dependable to hit upon the deleterious effect of missense mutations (Hinkle et al., 2003)
The degree of conservation of a particular position in a protein was determined using sequence homology based tool SIFT (Ng et al., 2003). The protein sequences of the 30 variants were submitted to SIFT to determine their tolerance indices. As the tolerance level increases, the functional influence of the amino acid substitution decreases and vice versa. Of the 12 variants, 6 variants seem deleterious, having tolerance index scores of ≤0.05 (Table I). Among these 6 variants, 5 variants showed a very high deleterious tolerance index score of 0.00 and one had a tolerance index score of 0.05 (Table I). Interestingly, 4 deleterious variants identified by SIFT were also found less stable by the I-Mutant2.0 server.
Table I: List of functionally significant mutants predicted to be by I-Mutant 2.0, SIFT and PolyPhen
Variant | ΔΔG | Tolerance index | PSIC SD |
---|---|---|---|
Q36L | -0.28 | 0 | 3.096 |
K41E | -2.61 | 0.6 | 0.13 |
K41I | -1.31 | 0.09 | 1.873 |
S52N | -3.92 | 0.5 | 0.616 |
R55K | -2.52 | 1 | 0.174 |
C63W | -0.59 | 0 | 4.091 |
K64I | 0.82 | 0 | 3.198 |
I70V | -0.59 | 0.36 | 0.259 |
K84E | -0.89 | 0.63 | 0.77 |
P136L | 0.67 | 0 | 3.436 |
V137I | -0.19 | 0.05 | 0.988 |
H138R | -1.16 | 0 | 3.419 |
Letters in bold indicate mutants predicted to be less stable, deleterious and damaging by I-Mutant2.0, SIFT and PolyPhen respectively |
Structural level alterations were worked out by PolyPhen program. Protein sequence with the position of mutation and the variants of amino acid linked with the 12 single point mutants were submitted to the PolyPhen server (Ramensky et al., 2002). A PSIC score difference of 1.1 and greater was regarded to be damaging. It could be known from Table I that, among the 12 variants, 6 were found damaging by PolyPhen. Also these variants showed a difference in PSIC score from 0.13 to 4.091. It was to be distinguished that the variants that were all found damaging by PolyPhen except for K41I were also identified as deleterious based on the SIFT server.
The 3 most potential detrimental point mutation were considered (Q36L, C63W and H138R) for further course of investigations because they were usually less stable, deleterious, and damaging by the I-Mutant2.0, SIFT and PolyPhen servers respectively (Capriotti et al., 2005; Ng et al., 2003; Ramensky et al., 2002). We considered the statistical accuracy of these three programs, I-Mutant improved the quality of the prediction of the free energy change caused by single point protein mutations by adopting a hypothesis of thermodynamic reversibility of the existing experimental data. The accuracy in prediction of sequence and structure based values were 78 and 84% with a correlation coefficient of 0.56 and 0.69, respectively (36). SIFT correctly predicted 69% of the substitutions associated with the disease that affect protein function. PolyPhen-2 evaluated rare alleles at loci potentially involved in complex phenotypes, densely mapped regions identified by genome-wide association studies, and analyzed natural selection of sequence data, where even mildly deleterious alleles must be treated as damaging. PolyPhen-2 was reported to achieve a rate of true positive predictions of 92% (Kumar et al., 2009; Capriotti et al., 2008; Ivan et al., 2010). To obtain precise and accurate measures of the detrimental effect of our variants, comprehensive parameters of all these three programs could be more significant than individual tool parameters. Figure 1 shows the pie chart distribution of functionally significant mutations based on SIFT, PolyPhen and I-Mutant2.0. Hence, we further investigated these detrimental missense mutations by structural analysis.
Figure 1: List of functionally significant mutations
Mapping the 3 variants namely, Q36L, C63W and H138R into the structural information of protein angiogenin was retrieved from SAAPdb. The structure accessible for angiogenin protein referred a PDB ID of 2ANG. The mutational position and amino acid variants were mapped in the native structure. Mutation at specified position was done by SWISSPDB viewer independently to obtain modeled structures. The energy minimization was then performed by the NOMAD - Ref server for both the native structure (PDB 2ANG) and mutant modeled structures. In order to find out the structural stability for angiogenin of native and mutants, we computed the total energy, which included bonds, angles, and torsions, non-bonded and electrostatic constraints from GROMOS 96 force field implemented in DeepView to check their stability. Table II showed that the total energy of the native protein had -10091.175KJ/mol whereas the 3 mutants had greater total energy than the native protein. The higher the total energy, lesser would be the protein structure stability. The native structure (PDB 2ANG) was superimposed with all the mutant modeled structures and RMSD calculated to find the deviation between the two structures. The higher the RMSD value, greater was the deviation between the native and mutant structure and this consecutively change the binding efficiency towards its interacting partners owing to deviation in the three dimensional space of the binding residues of angiogenin. RMSD values for native structure with all the mutant modeled structures were shown in Table II. Thus, Table II confirmed that, all the 3 mutants displayed an RMSD value ≥1.37Å. Figure 2 showed the super imposed structures of native with mutants (A) Q36L, (B) C63W and (C) H138R.
Figure 2: Superimposed structure of the native protein (green) with mutant (A) Superimposed structure of native angiogenin (green) with mutant Q36L (red) structure showing RMSD of 1.27 Ǻ. (B) Superimposed structure of native angiogenin (green) with mutant C63W (blue) structure showing RMSD of 1.37 Ǻ. (C) Superimposed structure of native angiogenin (green) with mutant H138R (magenta) structure showing RMSD of 1.20 Ǻ.
We further validated the stability of protein structure by using the PIC server to identify the number of intramolecular interactions of both the native and mutant structures. To understand the molecular root of stability, functions and the complexes of protein, the intra protein structure interactions and the inter protein structure interactions in a congregation were considered important. Several weak and strong intramolecular interactions that render stability of a protein structure were studied. Therefore these intramolecular interactions were computed by the PIC server in order to additionally validate the protein structure stability. Regarding this investigation, it was observed that totally 392 intramolecular interactions were obtained in the native structure of angiogenin. On the other hand, 3 mutant structures of Angiogenin established the intramolecular interactions between the range of 358 to 385 as shown in Table II. We further evaluated the effect of these 3 detrimental missense mutations by performing binding analysis between angiogenin and ribonuclease Inhibitor complex through protein-protein docking studies in order to understand the functional activity of angiogenin.
In order to find out the binding efficiency of native and mutant angiogenin with its interacting partner ribonuclease inhibitor, we implemented molecular dynamics approach for rationalizing the functional activity of these 3 mutants. In this analysis, we performed 3 missense mutations (Q36L, C63W and H138R) in the chain A of the PDB IDs 2ANG and 1A4Y by swisspdb viewer independently and energy minimization was performed for the entire complex (both native and mutant complex) by GROMACS (Nomad-ref). In order to obtain the optimized structures simulated annealing was done using a discrete molecular dynamics approach (ifold). We used GrammX to dock 2ANG native and mutant structures with 1A4Y and furthermore; we used DFire, for ï¬nding the protein conformation free energy source for the docked complex retrieved from GrammX. We used this server for the missense mutation analysis with respect to finding the free energy source of both native and mutants of angiogenin with ribonuclease inhibitor. In this analysis, we found that the binding free energy for ribonuclease inhibitor with native angiogenin protein was found to be -1215.6 kcal/mol, which had a higher binding affinity compared to the mutants. This analysis portrayed that native angiogenin exhibited higher binding affinity with ribonuclease inhibitor. Hence, the lesser binding free energies may probably be due to loss of intermolecular non-covalent interactions. This analysis clearly portrayed that the native complex had high intermolecular non covalent interactions than mutant complexes. Figure 3 showed the docked complex of ribonuclease inhibitor with (A) native angiogenin (B) Q36L, (C) C63W and (D) H138R.
Figure 3: Docked complexes of native and mutant angiogenin with ribonuclease inhibitor (A) Docked complex of Native Angiogenin (green) and Ribonuclease inhibitor (grey) having the Free energy of -1215.59, (B) Docked complex of Q36L (red) and Ribonuclease inhibitor (grey) having the Free energy of -1203.83, (C) Docked complex of C63W (blue) and Ribonuclease inhibitor (grey) having the Free energy of -1202.48, (D) Docked complex of H138R (magenta) and Ribonuclease inhibitor (grey) having the Free energy of -1198.17
To understand the cause of the lower substrate binding efficiency of the 3 detrimental missense mutation, the program ElNemo was used for comparing the amino acids flexibility that was involved in binding with ribonuclease inhibitor of both the native protein and the mutants. Table III and depicted the amino acids flexibility in the substrate binding pocket (active site) of both the native and mutant proteins through the normalized mean square displacement <R2>. These data were further categorized into three terms of flexibility. The first category was where the <R2> of the amino acids in the substrate binding pocket of the mutant was the same as that of the native protein (termed identical flexibility). The second category was where the <R2> of the amino acids in the substrate binding pocket of the mutant was higher than that of the native protein (termed increased flexibility). The last category was where the <R2> of the amino acids in the substrate binding pocket of a mutant was lower than that of the native protein (termed decreased flexibility). From the above investigation, we observed the increased flexibility of the substrate binding amino acids of these 3 mutants (Table III). Figure 4 showed the fluctuation of flexibility in both the native and mutant structures. Thus, most of the amino acids lost their flexibility during substrate binding of these mutants and thus they lost their binding efficiency with the substrate. Thus, this study showed that, increased flexibility of the protein was the cause for the loss in substrate binding affinity.
Figure 4: Comparison of normalized mean square displacement of binding amino acids of native and mutant angiogenin protein
Table II:RMSD, total energy, number of intramolecular interactions and free energy for the native protein and mutants
Variant | RMSD (Ã…) | Total energy (KJ/mol) | Total number of intra-molecular interactions | Free energy (Kcal/mol) |
---|---|---|---|---|
Native | 0.00 Ã… | -10091.175 | 392 | -1215.59 |
Q36L | 1.27 Ã… | -9249.535 | 385 | -1203.83 |
C63W | 1.37 Ã… | -9434.581 | 385 | -1202.48 |
H138R | 1.20 Ã… | -9677.100 | 358 | -1198.17 |
RMSD (root mean square deviation), total energy, total number of intra-molecular interactions and free energy |
Table III: Substrate binding amino acids of mutants with different ranges of flexibility based on<R2>
Variant | A | B | C |
---|---|---|---|
<R2> | <R2> | <R2> | <R2> |
Q36L | 8 | 12 | 0 |
C63W | 8 | 12 | 0 |
H138R | 7 | 13 | 0 |
Where the letter ‘A’ denotes amino acids with decreased flexibility of mutants than native; ‘B’ denotes amino acids with increased flexibility of both native and mutants; ‘C’ denotes amino acids with identical flexibility of both native and mutants |
Conclusion
By sequence and structure based approaches, we identified three variants (Q36L, C63W and H138R) were considered to be most detrimental point mutations causing Amyotrophic lateral sclerosis.
References
Adzhubei IA, Schmidt S, Peshkin L, Ramensky VE, Gerasimova A, Bork P, Kondrashov AS, Sunyaev SR. A method and server for predicting damaging missense mutations. Nat Methods. 2010; 7: 248-49.
Baker M, Mackenzie IR, Pickering-Brown SM, Gass J, Rademakers R, Lindholm C, Snowden J, Adamson J, Sadovnick AD, Rollinson S, Cannon A, Dwosh E, Neary D, Melquist S, Richardson A, Dickson D, Berger Z, Eriksen J, Robinson T, Zehr C, Dickey CA, Crook R, McGowan E, Mann D, Boeve B, Feldman H, Hutton M. Mutations in progranulin cause tau-negative frontotemporal dementia linked to chromosome 17. Nature 2006; 442: 916-19.
Bava KA, Gromiha MM, Uedaira H, Kitajima K, Sarai A. ProTherm, version 4.0: Thermodynamic database for proteins and mutants. Nucleic Acids Res. 2004; 32: D120-21.
Bento-Abreu A, Van Damme P, Van Den Bosch L, Robberecht W. The neurobiology of amyotrophic lateral sclerosis. Eur J Neurosci. 2010; 31: 2247-65.
Berman HM, Westbrook J, Feng Z, Gilliland G, Bhat TN, Weissig H, Shindyalov IN, Bourne PE. The Protein Data Bank. Nucleic Acids Res. 2000; 28: 235-42.
Boeckmann B, Bairoch A, Apweiler R, Blatter MC, Estreicher A, Gasteiger E, Martin MJ, Michoud K, O'Donovan C, Phan I, Pilbout S, Schneider M. The SWISS-PROT protein knowledgebase and its supplement TrEMBL in 2003. Nucleic Acids Res. 2003; 31: 365-70.
Bruijn LI, Miller TM, Cleveland DW. Unraveling the mechanisms involved in motor neuron degeneration in ALS. Annu Rev Neurosci. 2004; 27: 723-49.
Capriotti E, Fariselli P, Casadio R. I-Mutant2.0: Predicting stability changes upon mutation from the protein sequence or structure. Nucleic Acids Res. 2005; 33: W306-10.
Capriotti E, Fariselli P, Rossi I. A three-state prediction of single point mutations on protein stability changes. BMC Bioinformatics. 2008; 26: 56-59.
Carlson HA, McCammon JA. Accommodating protein flexibility in computational drug design. Mol Pharmacol. 2000; 57: 213-18.
Cavallo A, Martin AC. Mapping SNPs to protein sequence and structure data. Bioinformatics 2005; 21: 1443-50.
DeJesus-Hernandez M, Mackenzie IR, Boeve BF, Boxer AL, Baker M, Rutherford NJ, Nicholson AM, Finch NA, Flynn H, Adamson J, Kouri N, Wojtas A, Sengdy P, Hsiung GY, Karydas A, Seeley WW, Josephs KA, Coppola G, Geschwind DH, Wszolek ZK, Feldman H, Knopman DS, Petersen RC, Miller BL, Dickson DW, Boylan KB, Graff-Radford NR, Rademakers R. Expanded GGGGCC hexanucleotide repeat in noncoding region of C9ORF72 causes chromosome 9p-linked FTD and ALS. Neuron 2011; 72: 245-56.
Delarue M, Dumas P. On the use of low-frequency normal modes to enforce collective movements in refining macromolecular structural models. Proc Natl Acad Sci USA. 2004; 101: 6957-62.
Dion PA, Daoud H, Rouleau GA. Genetics of motor neuron disorders: New insights into pathogenic mechanisms. Nat Rev Genet. 2009; 10: 769-82.
Greenway MJ, Alexander MD, Ennis S, Traynor BJ, Corr B, Frost E, Green A, Hardiman O. A novel candidate region for ALS on chromosome 14q11.2. Neurology 2004; 63: 1936-38.
Hallahan TW, Shapiro R, Strydom DJ, Vallee BL. Importance of asparagine-61 and asparagine-109 to the angiogenic activity of human angiogenin. Biochemistry 1992; 31: 8022-29.
Hallahan TW, Shapiro R, Vallee BL. Dual site model for the organogenic activity of angiogenin. Proc Natl Acad Sci USA. 1991; 88: 2222-26.
Han JH, Kerrison N, Chothia C, Teichmann SA. Divergence of interdomain geometry in two-domain proteins. Structure 2006; 14: 935-45.
Hinkle A, Tobacman LS. Folding and function of the troponin tail domain, effects of cardiomyopathic troponin T mutations. J Biol Chem. 2003; 278: 506-13.
Kieran D, Sebastia J, Greenway MJ, King MA, Connaughton D, Concannon CG, Fenner B, Hardiman O, Prehn JH. Control of motoneuron survival by angiogenin. J Neurosci. 2008; 28: 14056-61.
Kishikawa H, Wu D, Hu GF. Targeting angiogenin in therapy of amyotropic lateral sclerosis. Expert Opin Ther Targets. 2008; 12: 1229-42.
Kobe B, Deisenhofer J. Mechanism of ribonuclease inhibition by ribonuclease inhibitor protein based on the crystal structure of its complex with ribonuclease. J Mol Biol. 1996; 264: 1028-43.
Kumar P, Henikoff S, Ng PC. Predicting the effects of coding non-synonymous variants on protein function using the SIFT algorithm. Nat Protoc. 2009; 4: 1073-81.
Lindahl E, Azuara C, Koehl P, Delarue M. NOMAD-Ref: visualization, deformation and refinement of macromolecular structures based on all-atom normal mode analysis. Nucleic acids Res. 2006; 34: 52-56.
Moroianu J, Riordan JF. Identification of the nucleolar targeting signal of human angiogenin. Biochem Biophys Res Commun. 1994; 203: 1765-72.
Ng PC, Henikoff S. Predicting deleterious amino acid substitutions. Genome Res. 2001; 11: 863-74.
Ng PC, Henikoff S. SIFT: Predicting amino acid changes that affect protein function. Nucleic Acids Res. 2003; 31: 3812-14.
Padhi AK, Kumar H, Vasaikar SV, Jayaram B, Gomes J. Mechanisms of loss of functions of human angiogenin variants implicated in amyotrophic lateral sclerosis. PLoS ONE. 2012; 7: e32479.
Parthasarathy S, Murthy MRN. Protein thermal stability: Insights from atomic displacement parameters (B values). Protein Eng. 2000; 13: 9-13.
Ramensky V, Bork P, Sunyaev S. Human non-synonymous SNPs: Server and survey. Nucleic Acids Res. 2002; 30: 3894-900.
Renton AE, Majounie E, Waite A, Simón-Sánchez J, Rollinson S, Gibbs JR, Schymick JC, Laaksovirta H, van Swieten JC, Myllykangas L, et al. A hexanucleotide repeat expansion in C9ORF72 is the cause of chromosome 9p21-linked ALS-FTD. Neuron 2011; 72: 257-68.
Ringe D, Petsko GA. Study of protein dynamics by X-ray diffraction. Methods Enzymol. 1986; 131: 389-433.
Shapiro R, Riordan JF, Vallee BL. Characteristic ribonucleolytic activity of human angiogenin. Biochemistry 1986; 25: 3527-32.
Shapiro R. Cytoplasmic ribonuclease inhibitor. Methods Enzymol. 2001; 341: 611-28.
Sharma S, Ding F, Nie H, Watson D, Unnithan A, Lopp J, Pozefsky D, Dokholyan NV. iFold: A platform for interactive folding simulation of proteins. Bioinformatics 2006; 22: 2693-94.
Subramanian V, Crabtree B, Acharya KR. Human angiogenin is a neuroprotective factor and amyotrophic lateral sclerosis associated angiogenin variants affect neurite extension/pathfinding and survival of motor neurons. Hum Mol Genet. 2008; 17: 130-49.
Suhre K, Sanejouand YH. ElNe´mo: A normal mode web-server for protein movement analysis and the generation of templates for molecular replacement. Nucleic Acids Res. 2004; 32: 610-14.
Tina KG, Bhadra R, Srinivasan N. PIC: Protein interactions calculator. Nucleic Acids Res. 2007; 35: W473-76.
Tovchigrechko A, Vakser IA. GRAMM-X public web server for protein-protein docking. Nucleic Acids Res. 2006; 34: 310-14.
Vande Velde C, Dion PA, Rouleau GA. Amyotrophic lateral sclerosis: New genes, new models, and new mechanisms. F1000 Biol Rep. 2011; 3: 18.
Varfolomeev SD, Uporov IV, Fedorov EV. Bioinformatics and molecular modeling in chemical enzymology: Active sites of hydrolases. Biochemistry (Mosc). 2002; 67: 1099-108.
Wu D, Yu W, Kishikawa H, Folkerth RD, Iafrate AJ, Shen Y, Xin W, Sims K, Hu GF. Angiogenin loss-of-function mutations in amyotrophic lateral sclerosis. Ann Neurol. 2007; 62: 609-17.
Yip YL, Famiglietti M, Gos A, Duek PD, David FP, Gateau A, Bairoch A. Annotating single amino acid polymorphisms in the UniProt/Swiss-Prot knowledge base. Hum Mutat. 2008; 29: 361-66.
Yip YL, Scheib H, Diemand AV, Gattiker A, Famiglietti LM, Gasteiger E, Bairoch A. The Swiss-Prot variant page and the ModSNP database: A resource for sequence and structure information on human protein variants. Hum Mutat. 2004; 23: 464-70.
Yuan Z, Bailey TL, Teasdale RD. Prediction of protein B-factor profiles. Proteins 2005; 58: 905-12.
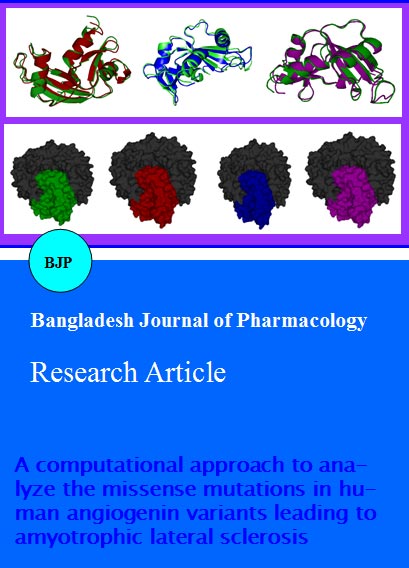