Discovery of potential cholesterol esterase inhibitors using in silico docking studies
Abstract
New drug discovery is considered broadly in terms of two kinds of investigational activities such as exploration and exploitation. This study deals with the evaluation of the cholesterol esterase inhibitory activity of flavonoids apigenin, biochanin, curcumin, diosmetin, epipervilline, glycitein, okanin, rhamnazin and tangeritin using in silico docking studies. In silico docking studies were carried out using AutoDock 4.2, based on the Lamarckian genetic algorithm principle. The results showed that all the selected flavonoids showed binding energy ranging between -7.1 kcal/mol to -5.6 kcal/mol when compared with that of the standard compound gallic acid (-4.1 kcal/mol). Intermolecular energy (-9.1 kcal/mol to -7.1 kcal/mol) and inhibition constant (6.5 µM to 73.2 µM) of the ligands also coincide with the binding energy. All the selected flavonoids contributed cholesterol esterase inhibitory activity, these molecular docking analyses could lead to the further development of potent cholesterol esterase inhibitors for the treatment of obesity
Introduction
Obesity is becoming one of the greatest threats to global health in this millennium, with more than 1 billion overweight adults and of those, at least 300 million are clinically obese. The obesity market reached US$ 3.7 billion by 2008 and has been predicted to reach US$ 6.1 billion by 2015. The mushrooming market for these drugs and the vast sum of money at stake guarantee that research in this therapeutic area will not slow down within the predictable (Birari and Bhutani, 2007). Obesity is resulting from an energy imbalance caused by increased ratio of caloric intake to energy expenditure and the excess body fats thus formed are stored in body. Obesity is also known to be risk factor for the development of metabolic disorders, dyslipidemia and atherosclerosis and type-2 diabetes. In recent years, there has been a great increase in the use of herbal medicines for the treatment of obesity (Ramgopal et al., 2010). Overweight and obesity are the fifth leading risk for global deaths. At least 2.8 million adults die each year as a result of being overweight or obese (Barness et al., 2007)
Pancreatic cholesterol esterase (CEase) is secreted from vertebrate pancreas into the intestinal track and activated by primary bile salts. It is present in few mammals including humans (John et al., 2010). CEase belongs to alpha/beta-hydrolase fold family. The active site includes both the catalytic triad (Ser-194, Asp-320, His-435) and oxyanion hole (Gly-107, Ala-108, Ala-195) residues (John et al., 2010). CEase catalyzes the hydrolys is of sterol esters into their component sterols and fatty acids. It is responsible for the hydrolysis of various substrates including dietary cholesterol esters, fat-soluble vitamins, triglycerides, and phospholipids. Flavonoids are widespread phytochemical constituents present in plants and they contribute the flavor and color of fruits and vegetables. They are 15 carbons of 2 phenolic rings connected by a 3-carbon unit and grouped according to presence of various functional groups on the rings and the degree of ring saturation (Whittern et al., 1984). They are usually attached with sugars moiety to increase their water-solubility. Most of the flavonoids are known to possess various pharmacological activities, such as anti-inflammatory activity (Funakoshi et al., 2011), neuroprotective effects (Datla et al., 2001), antibacterial (Martini et al., 2001; Kil et al., 2012), antioxidant (Pande, 2004), antiproliferative (Harinantenaina et al., 2010), cytostatic effect (Androutsopoulos et al., 2012), and antiviral (Chen et al., 2010).
Nowadays, the use of computers to predict the binding of libraries of small molecules to known target structures is an increasingly important component of the drug discovery process (Shoichet, 2004; Koppen, 2009). There is a wide range of software packages available for the conduct of molecular docking simulations like, AutoDock and DOCK, GOLD, FlexX and ICM (Collignon et al., 2011). AutoDock 4.2 is the most recent version which has been widely used for virtual screening, due to its enhanced docking speed (Schames et al., 2004). Its default search function is based on Lamarckian Genetic Algorithm (LGA), a hybrid genetic algorithm with local optimization that uses a parameterized free-energy scoring function to estimate the binding energy. Each docking is comprised of multiple independent executions of LGA and a potential way to increase its performance is to parallelize the aspects for execution (Cosconati et al., 2010).
The stereochemistry of flavonoids binding on CEase has not been characterized. In this study, the search of flavonoids in the molecular basis for binding to active site of CEase is revealed by computer aided docking analysis.
Materials and Methods
Softwares required
Python 2.7-language was downloaded from www.python.com, Cygwin (a data storage) c:\program and Python 2.5 were simultaneously downloaded from www.cygwin.com, Molecular graphics laboratory (MGL) tools and AutoDock 4.2 was downloaded from www.scripps.edu, Discovery studio visualizer 2.5.5 was downloaded from www.accelerys.com, Molecular orbital package (MOPAC), Chemsketch was downloaded from www.acdlabs.com. Online smiles translatory notation was carried out using cactus.nci.nih.gov/translate/.
Coordinate file preparation
An extended PDB format, termed as PDBQT file was used for coordinate files which includes atomic partial charges. AutoDock Tools was used for creating PDBQT files from traditional PDB files (Khodade et al., 2007). Crystal structure of CEase (PDB ID: 1F6W) was downloaded from the RCSB protein data bank (Figure 1).
Figure 1: Cholesterol esterase enzyme from RCSB (1F6W)
In Figure 2, the flavonoid ligands like apigenin, biochanin, curcumin, diosmetin, epipervilline, glycitein, okanin, rhamnazin, tangeritin and the standard gallicacid were built using Chemsketch and optimized using “Prepare Ligands†in the AutoDock 4.2 for docking studies. The optimized ligand molecules were docked into refined CEase model using "LigandFit" in the AutoDock 4.2 (Goodsell et al., 1996).
Figure 2: The optimized ligand molecules (1. Apigenin, 2. Biochanin, 3. Curcumin, 4. Diosmetin, 5. Epipervilline, 6. Glycitein, 7. Okanin, 8. Rhamnazin, 9. Tangeritin and 10. Gallicacid)
AutoGrid calculation
Rapid energy evaluation was achieved by precalculating atomic affinity potentials for each atom in the ligand molecule. In the AutoGrid procedure, the target enzyme was embedded on a three dimensional grid point (Morris et al., 1998). The energy of interaction of each atom in the ligand was encountered.
AutoDock calculation
Docking can be carried out by various methods. But, the most efficient method is Lamarckian genetic algorithm. AutoDockwas run several times to getvarious docked conformations, and used to analyzethe predictted docking energy. The binding sites for these molecules were selected based on the ligand-binding pocket of the templates (Chang et al., 2010).
Analysis using AutoDock Tools
AutoDock Tools provide various methods to analyze the results of docking simulations such as, conformational similarity, visualizing the binding site and its energy and other parameters like intermolecular energy and inhibition constant. For each ligand, ten best poses were generated and scored using AutoDock 4.2 scoring functions (Park et al., 2006).
Result and Discussion
The docking poses were ranked according to their docking scores and both the ranked list of docked ligands and their corresponding binding poses (Zhang et al., 2008). In Figure 3, docked pose of CEase enzyme with epipervilline ligand clearly demonstrated the binding positions of the ligand with the enzyme. Binding energy of the individual compound were calculated using the following formula:
Figure 3: Docked pose of cholesterol esterase enzyme (1F6W) with Epipervilline
Binding energy = A + B + C - D
Where, A denotes final intermolecular energy + Wander valls energy (vdW) + hydrogen bonds + desolvation energy + electrostatic energy (kcal/mol), B denotes final total internal energy (kcal/mol), C denotes torsional free energy (kcal/mol), D denotes unbound system’s energy (kcal/mol)
In the Figure 3, the binding sites of the most active flavonoid Epipervilline was cleary showed and it was found to be, GLY-106, GLY-107, ALA-108, TYR-125, GLU-193, SER-194, TRP-227, VAL-285, LEU-282, PHE-324, LEU-392, PHE-393, HIS-435 and ILE-439 .
In Figure 4, the potential binding sites of the standard was clearly showed and it was found that, ALA-117, ASN-118, PHE-119, ASN-121, ASN-122, TYR-123, LEU-124, LYS-445. This proves that the effective binding sites are present in the selected flavonoid epipervilline when compared with the standard gallic acid. It proves that the ability of inhibiting the CEase enzyme by the selected flavonoid.
Figure 4: Docked pose of cholesterol esterase enzyme (1F6W) with gallic acid
Analysis of the receptor/ligand complex models generated after successful docking of the flavonoids was based on the parameters such as, hydrogen bond interactions, drug-drug interactions, binding energy, RMSD of active site residues and orientation of the docked compound within theactive site. As a general rule, in most of the potent antigout compounds, both hydrogen bond and drug-drug hydrophobic interactions between the compound and the active sites of the receptor have been found to be responsible for mediating the biological activity (Umamaheswari et al., 2011).
As shown in Table I, flavonoids showed binding energy ranging between -7.1 to -5.6 kcal/mol. All the selected flavonoids had lesser binding energy when compared to the standard gallic acid (-4.1 kcal/mol). This proves
 that flavonoids consist of potential CEase inhibitory binding sites when compared to the standard. In addition, two other parameters like inhibition constant (Ki) and intermolecular energy were also determined. As shown in Table II, flavonoids showed inhibition constant ranging from 6.5 to 73.2 µM. All the selected compounds had lesser inhibition constant when compared to the standard (357.8 µM). Inhibition constant is directly proportional to binding energy. We found a decrease in inhibition constant of all the selected flavonoids with a simultaneous decrease in the binding energy. Thus, the cholesterol esterase inhibitory activity of the flavonoids were found to be higher compared to gallic acid.
Table I: Binding energies of the compounds based on their rank
Compound | Binding energies of the compounds based on their rank (kcal/mol-1) | |||||||||
---|---|---|---|---|---|---|---|---|---|---|
1 | 2 | 3 | 4 | 5 | 6 | 7 | 8 | 9 | 10 | |
Apigenin | -5.9 | -5.9 | -5.7 | -5.7 | -5.7 | -5.7 | -5.7 | -5.7 | -5.7 | -5.7 |
Biochanin | -6.5 | -6.5 | -6.5 | -6.5 | -6.5 | -6.5 | -6.5 | -6.5 | -6.5 | -6.4 |
Curcumin | -6.1 | -5.1 | -5.5 | -5.0 | -5.0 | -5.0 | -5.3 | -5.1 | -5 | -4.8 |
Diosmetin | -6.0 | -6.0 | -6 | -6.0 | -6.0 | -5.9 | -5.9 | -5.9 | -5.8 | -5.8 |
Epipervilline | -7.1 | -7.1 | -7.1 | -7.1 | -7.1 | -7.1 | -7.0 | -7.0 | -6.6 | -6.5 |
Glycitein | -6.1 | -5.5 | -5.5 | -5.5 | -5.4 | -5.4 | -5.4 | -5.3 | -5.3 | -5.2 |
Okanin | -6.4 | -5.4 | -5.3 | -5.0 | -5.0 | -4.6 | -4.5 | -5.1 | -4.9 | -4.8 |
Rhamnazin | -5.6 | -5.6 | -5.2 | -5.0 | -4.9 | -4.6 | -4.4 | -4.8 | -4.5 | -4.8 |
Tangeritin | -6.1 | -5.9 | -5.6 | -5.6 | -5.4 | -5.3 | -5.5 | -5.3 | -5.1 | -5.1 |
Gallic acid | -4.1 | -4.1 | -4.1 | -4.1 | -4.1 | -4.1 | -4.1 | -4.1 | -4.1 | -4.0 |
Table II: Inhibition constant of the compounds based on their rank
Compound | Intermolecular energies of the compounds based on their rank (kcal/mol) | |||||||||
---|---|---|---|---|---|---|---|---|---|---|
1 | 2 | 3 | 4 | 5 | 6 | 7 | 8 | 9 | 10 | |
Apigenin | -7.1 | -7.1 | -6.9 | -6.9 | -6.9 | -6.9 | -6.9 | -6.9 | -6.9 | -6.9 |
Biochanin | -7.7 | -7.7 | -7.7 | -7.7 | -7.7 | -7.7 | -7.7 | -7.7 | -7.7 | -7.6 |
Curcumin | -9.1 | -8.1 | -8.5 | -8 | -8.0 | -8.0 | -8.3 | -8.1 | -8.0 | -6.9 |
Diosmetin | -7.5 | -7.5 | -7.5 | -7.5 | -7.5 | -7.4 | -7.4 | -7.4 | -7.3 | -7.3 |
Epipervilline | -8.0 | -8.0 | -8.0 | -8.0 | -7.9 | -7.9 | -7.9 | -7.9 | -7.5 | -7.4 |
Glycitein | -7.3 | -6.7 | -6.7 | -6.7 | -6.6 | -6.6 | -6.6 | -6.5 | -6.5 | -6.3 |
Okanin | -8.8 | -7.8 | -7.7 | -7.4 | -7.4 | -7.0 | -6.9 | -7.5 | -7.3 | -7.1 |
Rhamnazin | -7.4 | -7.4 | -7.0 | -6.8 | -6.7 | -6.4 | -6.2 | -6.6 | -6.2 | -6.6 |
Tangeritin | -7.9 | -7.7 | -7.4 | -7.4 | -7.2 | -7.1 | -7.3 | -7.0 | -6.9 | -6.9 |
Gallic acid | -6.2 | -6.2 | -6.0 | -5.9 | -5.9 | -5.6 | -5.6 | -6.0 | -5.8 | -5.6 |
As shown in Table III, flavonoids showed inter-molecular energy ranging between -9.1 to -7.1 kcal/mol which was lesser when compared to the standard (-6.2 kcal/mol). Intermolecular energy is also directly proportional to binding energy. We found a decrease in intermolecular energy of all the selected compounds with a simultaneous decrease in the binding energy. This result further proved the CEase inhibitory activity of all the selected flavonoids.
Table III: Intermolecular energies of the compounds based on their rank
Compound | Intermolecular energies of the compounds based on their rank (kcal/mol) | |||||||||
---|---|---|---|---|---|---|---|---|---|---|
1 | 2 | 3 | 4 | 5 | 6 | 7 | 8 | 9 | 10 | |
Apigenin | -7.1 | -7.1 | -6.9 | -6.9 | -6.9 | -6.9 | -6.9 | -6.9 | -6.9 | -6.9 |
Biochanin | -7.7 | -7.7 | -7.7 | -7.7 | -7.7 | -7.7 | -7.7 | -7.7 | -7.7 | -7.6 |
Curcumin | -9.1 | -8.1 | -8.5 | -8 | -8.0 | -8.0 | -8.3 | -8.1 | -8.0 | -6.9 |
Diosmetin | -7.5 | -7.5 | -7.5 | -7.5 | -7.5 | -7.4 | -7.4 | -7.4 | -7.3 | -7.3 |
Epipervilline | -8.0 | -8.0 | -8.0 | -8.0 | -7.9 | -7.9 | -7.9 | -7.9 | -7.5 | -7.4 |
Glycitein | -7.3 | -6.7 | -6.7 | -6.7 | -6.6 | -6.6 | -6.6 | -6.5 | -6.5 | -6.3 |
Okanin | -8.8 | -7.8 | -7.7 | -7.4 | -7.4 | -7.0 | -6.9 | -7.5 | -7.3 | -7.1 |
Rhamnazin | -7.4 | -7.4 | -7.0 | -6.8 | -6.7 | -6.4 | -6.2 | -6.6 | -6.2 | -6.6 |
Tangeritin | -7.9 | -7.7 | -7.4 | -7.4 | -7.2 | -7.1 | -7.3 | -7.0 | -6.9 | -6.9 |
Gallic acid | -6.2 | -6.2 | -6.0 | -5.9 | -5.9 | -5.6 | -5.6 | -6.0 | -5.8 | -5.6 |
Based on the docking studies, the CEase inhibitory activity of the selected compounds was found to be decreased in the order of epipervilline, biochanin, okanin, curcumin, tangeritin, glycitein, diosmetin, apigenin, rhamnazinand gallic acid. On the basis of the above study, all the selected compounds possess potential CEase inhibitory binding sites when compared to that of the standard. This may be attributed due to the differences in the position of the functional groups in the compounds. Flavonoids consist of benzopyran ring in its basic nucleus which could be responsible for the CEase inhibitory activity.
These results clearly indicate that flavonoids especially, Epipervilline possess potential CEase inhibitory binding sites and further investigations on the above compounds are necessary to develop potential chemical entities for the prevention and treatment of obesity and related disorders.
References
Androutsopoulos VP, Spandidos DA. The flavonoids diosmetin and luteolin exert synergistic cytostatic effects in human hepatoma HepG2 cells via CYP1A-catalyzed metabolism, activation of JNK and ERK and P53/P21 up-regulation. J Nutr Biochem. 2012; 6: 27.
Barness LA, Opitz JM, Gilbert-Barness E. Obesity: Genetic, molecular, and environmental aspects. Am J Med Genet A. 2007; 143A: 3016-34.
Birari RB, Bhutani KK. Pancreatic lipase inhibitors from natural sources: Unexplored potential. Drug Discov Today. 2007; 12: 879-89.
Chang MW, Ayeni C, Breuer S, Torbett BE. Virtual screening for HIV protease inhibitors: A comparison of AutoDock 4 and vina. PLoS One. 2010; 5: e11955.
Collignon B, Schulz R, Smith JC, Baudry J. Task-parallel message passing interface implementation of AutoDock 4 for docking of very large databases of compounds using high-performance super-computers. J Comput Chem. 2011; 32: 1202-09.
Cosconati S, Forli S, Perryman AL, Harris R, Goodsell DS, Olson AJ. Virtual Screening with AutoDock: Theory and practice. Expert Opin Drug Discov. 2010; 5: 597-607.
Chen DY, Shien JH, Tiley L, Chiou SS, Wang SY, Chang TJ, Lee YJ, Chan KW, Hsu WL. Curcumin inhibits influenza virus infection and haemagglutination activity. Food Chem. 2010; 119: 1346-51.
Datla K, Christidou M, Widmer WW, Rooprai HK, Dexter DT. Tissue distribution and neuroprotective effects of citrus flavonoid tangeretin in a rat model of Parkinson's disease. Neuroreport 2001; 12: 3871-75.
Goodsell DS, Morris GM, Olson AJ. Automated docking of flexible ligands: Applications of AutoDock. J Mol Recognit. 1996; 9: 1-5.
Harinantenaina L, Brodie PJ, Slebodnick C, Callmander MW, Rakotobe E, Randrianasolo S, Randrianaivo R, Rasamison VE, Tendyke K, Shen Y, Suh EM, Kingston DG. Antiproliferative compounds from Pongamiopsis pervilleana from the Madagascar Dry Forest. J Nat Prod. 2010; 73: 1559-62.
Kil JS, Son Y, Cheong YK, Kim NH, Jeong HJ, Kwon JW, Lee EJ, Kwon TO, Chung HT, Pae HO. Okanin, a chalcone found in the genus Bidens, and 3-penten-2-one inhibit inducible nitric oxide synthase expression via heme oxygenase-1 induction in RAW264.7 macrophages activated with lipopolysaccharide. J Clin Biochem Nutr. 2012; 50: 53-58.
John ST, Sakkiah S, Lee KW. Discovery of potential pancreatic cholesterol esterase inhibitors using pharmacophore modelling, virtual screening and optimization studies. J Enzyme Inhib Med Chem. 2011; 26: 535-45.
Khodade P, Prabhu R, Chandra N, Raha S, Govindarajan R. Parallel implementation of AutoDock. J Appl Cryst. 2007; 40: 598-99.
Koppen H. Virtual screening – what does it give us? Curr Opin Drug Discov Devel. 2009; 12: 397-407.
Martini ND, Katerere DR, Eloff JN. Biological activity of five antibacterial flavonoids from Combretumery throphyllum(Combretaceae). Pharmazie 2001; 56: 749-50.
Morris GM, Goodsell DS, Halliday RS Huey R, Hart WE, Belew RK, Olson AJ. Automated docking using Lamarckian genetic algorithm and an empirical binding free energy function. J Comput Chem. 1998; 19: 1639-62.
Morris GM, Huey R, Lindstrom W, Sanner MF, Belew RK, Goodsell DS, Olson AJ. AutoDock 4 and AutoDock Tools 4: Automated docking with selective receptor flexibility. J Comput Chem. 2009; 30: 2785-91.
Pande V. Antioxidant activity of rhamnazin-4-O-beta-[apiosyl(1-2)] glucoside in the brain of aged rats. Die Pharmazie. 2001; 56: 749-50.
Park H, Lee J, Lee S. Critical assessment of the automated AutoDock as a new docking tool for virtual screening. Proteins 2006; 65: 549-54.
Ramgopal M, Attitalla IH, Avinash P, Balaji M. Evaluation of antilipidemic and anti-obesity efficacy of Bauhinia purpurea bark extract on rats fed with high fat diet. Academic J Plant Sci. 2010; 3: 104-07.
Schames JR, Henchman RH, Siegel JS, Sotriffer CA, Ni H, McCammon JA. Discovery of a novel binding trench in HIV integrase. J Med Chem. 2004; 47: 1879-81.
Shoichet BK. Virtual screening of chemical libraries. Nature 2004; 432: 862-65.
Umamaheswari M, Madeswaran A, Asokkumar K, Sivashanmugam T, Subhadradevi V, Jagannath P. Discovery of potential xanthine oxidase inhibitors using in silico docking studies. Der Pharma Chemica. 2011; 3: 240-47.
Whittern CC, Miller EE, Pratt DE. Cottonseed flavonoids as lipid antioxidants. J Am Oil Chem Soc. 1984; 61: 1075-78.
Zhang S, Kumar K, Jiang X, Wallqvist A, Reifman J. DOVIS: An implementation for high throughput virtual screening using AutoDock. BMC Bioinformatics. 2008; 9: 126.
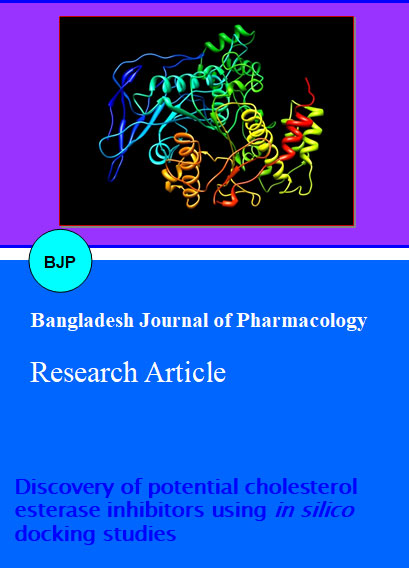